News
How to More Closely Intertwine Clinicians and Their Algorithms in Patient Care
Ravi Parikh Eyes Futuristic Concepts Combining AI and Physicians as True Partners
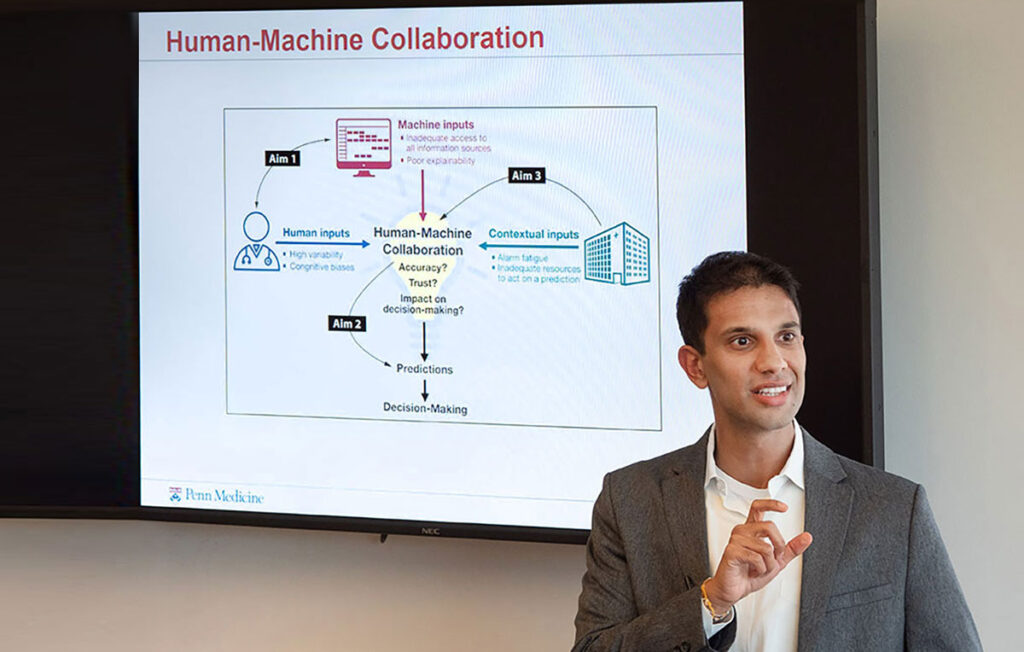
LDI Associate Fellow Ravi Parikh is thinking about futuristic concepts combining AI and physicians as true partners. He was the featured speaker at the inaugural seminar of Abramson Cancer Center’s new Penn Center for Cancer Care Innovation (PC3I).
What are the issues raised when a clinician uses information produced by artificial intelligence to treat a patient when it’s not known exactly how that machine arrived at that solution — because its algorithms are black boxes of incomprehensible computer code? This kind of “unexplainability” in machine learning decision support systems is just one of the issues confronting researchers exploring how to pair the most advanced artificial intelligence systems with human clinicians in ways that constitute true collaboration between the two.
Speaking at the inaugural seminar of the Abramson Cancer Center’s new Penn Center for Cancer Care Innovation (PC3I), Ravi Parikh, MD, MPP, discussed his research thinking in this nascent field of human-machine clinical collaborations. The talk was titled “Human-Machine Collaborations to Predict Mortality in Cancer.”
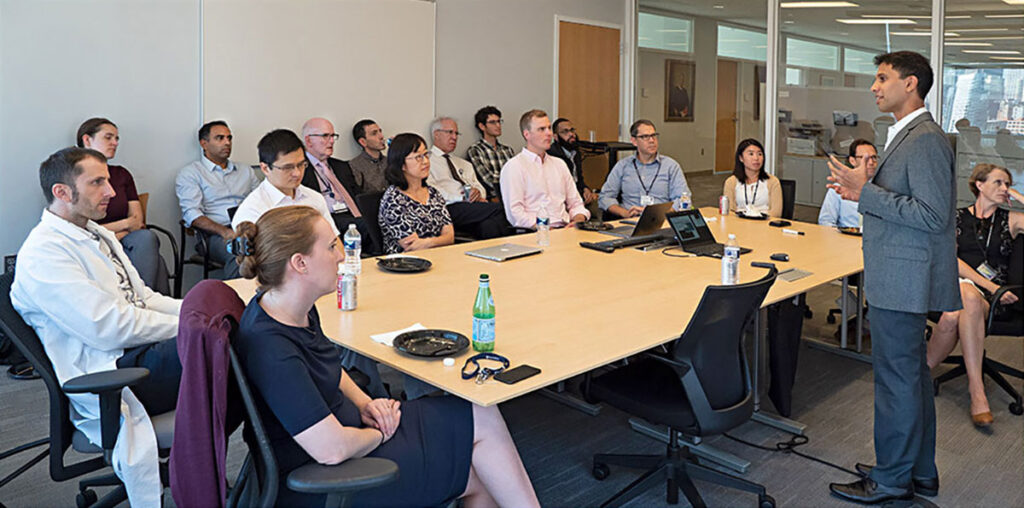
Parikh, an Instructor in the Penn Medicine Department of Medical Ethics and Health Policy, a PC3I innovation faculty member, and an Associate Fellow at Penn’s Leonard Davis Institute of Health Economics (LDI).
Machine/human collaborations
“I’m looking at methodology to measure the added value of human/machine collaborations in clinical decision-making using the case example of predicting mortality in cancer,” Parikh told the Penn Medicine gathering.
A long-time quandary for clinicians providing care to patients with cancer is estimating how much longer an individual patient will live. That prognosis is a crucial element for determining appropriate treatment strategies as well as supporting other end-of-life decisions.
But because physicians tend not to be very good at this kind of mortality estimating, a number of research projects have been working to develop algorithmic systems able to more accurately predict mortality in seriously ill patients.
Combining unique insights
What makes Parikh’s research project so interesting is that instead of viewing the algorithm as a dumb mathematical tool that human clinicians use like a calculator to augment their own thinking, he is studying how the most advanced machine learning systems might work as true partners with humans. The idea being that both parties — human and machine — develop unique insights into a patient’s condition and care to collaboratively reach the decisions most likely to improve outcomes. Or, in this area of his immediate focus, more accurately predict a terminal patient’s remaining length of life.
“In one randomized trial, we’re thinking about the AI as a sort of advanced decision support tool,” said Parikh. “But there are also a host of other ways we could think about that. For example, we could think of systems where the algorithm uses human inputs to revise and adjust its predictions, coming up with real human/machine interactive predictions. That’s the current basis of our conceptual model for human-machine collaboration. The question being asked is how do we measure these kinds of collaborative interactions in a rigorous way?”
On screen behind Parikh was an illustration mapping out the difficult challenges to be solved in such work, including the machine’s inadequate access to all information sources or the human clinician’s high variability and cognitive biases.
“Some of the literature and the popular press have focused on how machine learning can outperform humans in diagnosing lung nodules as lung cancers and things like that,” said Parikh. “What I think needs to be done is to just figure out what humans are good at and what machines are good at, because it’s likely those are two different things.”
The Deep Blue effect
Parikh noted that the issue of AI versus human intelligence became a major populist interest in 1997 when World Chess Champion Garry Kasparov was defeated by Deep Blue, an IBM supercomputer. It was the first time a machine had beaten a world chess champion in tournament conditions. That achievement sparked long-running discussions and debates about the potential superiority of AI in a variety of industries, including health care.
However, subsequent studies have found that when a two-part team of algorithm and human were functioning collaboratively, they repeatedly beat the straight supercomputer algorithm at chess.
“I think this illustrates the potential of these tools,” said Parikh. “No one in their right mind is thinking about setting future algorithms loose to dictate decisions for the patient. In some way, there has to be a human-machine system that is responsible for deploying these algorithms and responsible for improving outcomes and we really haven’t thought about what that optimal human-machine system looks like.”