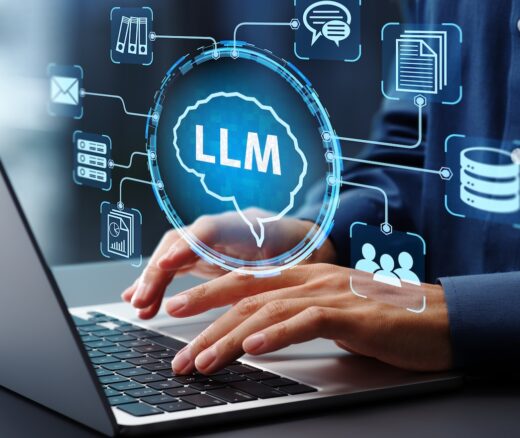
AI in Health Care and the FDA’s Blind Spot
A Novel Study Shows That Large Language Models Act Like Medical Devices in Clinical Care Scenarios
Blog Post
Assessments of likely cancer outcomes are complicated and often involve some degree of guesswork. Research has shown that oncologists—like other doctors—tend to overestimate the prognoses of their patients. Overoptimistic prognoses may be rooted in good intentions, but they can cause harm. They lead to overly aggressive therapy, fewer appropriate referrals to hospice, and a delay in advanced care planning.
Machine learning algorithms hold promise to improve prognostication. They can identify patients who are at risk of either dying or having significant declines. Furthermore, machine learning algorithms can be integrated into routine “nudges” for clinicians to increase rates of critical conversations and advanced care planning among high-risk patients.
Little is known, however, about how oncologists perceive the integration of machine learning prognoses into their practice. To learn more, my coauthors and I conducted qualitative interviews with practicing oncology physicians and advance practice providers. We interviewed 29 oncology clinicians (19 physicians, 10 APPs) across the University of Pennsylvania Health System about their views on the potential utility of machine learning prognostic algorithms for their practice—and their concerns about them.
As we reported recently in Supportive Care in Cancer, we found that clinicians believe such algorithms hold utility in practice, particularly to prompt end-of-life conversations. As one physician said: “It would serve more of a reminder to me, like, ‘Hey, maybe it’s time to have this conversation.’ I’m well aware that these things are impossible to predict, but to me it would be maybe a prompt because sometimes us physicians can even fool ourselves to thinking that things are okay when they’re not.”
However, in addition to seeing the benefits of machine learning prognoses, clinicians also had several concerns. They worried about the accuracy of algorithmic predictions and expressed concern that clinicians would rely on machine predictions more than clinical intuition. As one clinician said: “It could make me less present or available to my patients, because [the algorithm] is doing my work for me.”
The clinicians also expressed ethical concerns about disclosing machine-generated prognoses to patients. In particular, they worried about patients discovering the prognoses in the electronic health record on their own without support from someone who could explain it.
The “black box” nature of current machine learning algorithms also presented problems. Clinicians know what variables matter most in a simple risk score, but much of that information is hidden in machine learning. More “explainable” artificial intelligence and machine learning may facilitate greater trust and move clinicians toward relying on algorithms that help improve end-of-life decision-making. However, the field of “explainable” machine learning is nascent. As machine learning integrates into clinical care and developers face a tough choice between transparent algorithms and more accurate “black box” algorithms, they will have to consider what will drive clinician behavior.
When we asked the clinicians about the potential with machine learning prognostication for false positive versus false negative predictions, we found no overt preference. The issue is important to the development of prognostication algorithms because false positives may generate too many alerts and cause clinicians to ignore the algorithm prompts while too many false negatives—that is, high-risk patients being overlooked by the algorithm—would also diminish trust. A promising strategy to encourage clinician buy-in is a system in which individual clinicians can essentially “turn the knob” to tailor how often or when predictions are generated. This approach opens new possibilities for human-machine collaboration in the field of end-of-life care. Another advantage to this flexible approach is that it would allow clinicians to adjust the algorithm to reflect a patient’s values.
This study, the first to explore the perspectives of oncology clinicians towards machine learning prognostication, opens the door for deeper research to advance the field of AI in medicine. Most notably, it highlights that how these tools are incorporated into physician-patient interactions is just as important as the programming behind predictive and diagnostic algorithms—findings that apply not only to oncologists, but to clinicians in many other specialties as well.
The study, Clinician Perspectives on Machine Learning Prognostic Algorithms in the Routine Care of Patients with Cancer: A Qualitative Study, was published in Supportive Care in Cancer in May 2022. Authors include Ravi B. Parikh, Christopher R. Manz, Maria N. Nelson, Chalanda N. Evans, Susan H. Regli, Nina O’Connor, Lynn M. Schuchter, Lawrence N. Shulman, Mitesh S. Patel, Joanna Paladino, and Judy A. Shea.
A Novel Study Shows That Large Language Models Act Like Medical Devices in Clinical Care Scenarios
A Penn Lab Takes a New Approach to Unpacking the Doctor-Patient Encounter
We Need a System That Watches AI Care at the Bedside, Two LDI Fellows Say
A New Study Adds More Clinical Factors to the Algorithmic Process
Machine-Learning Tool Combined with Behavioral Nudges Scaled Down Treatment While Maintaining Supportive Therapies
An interview with Penn LDI Senior Fellow Hummy Song