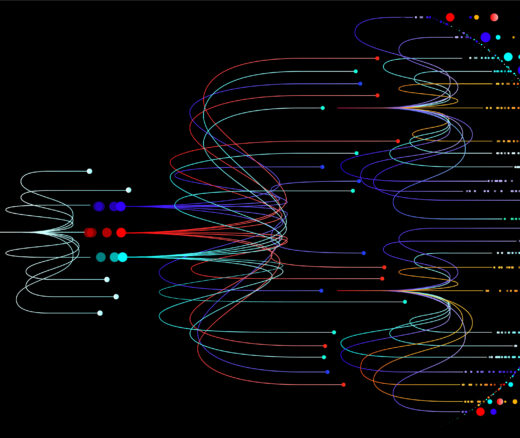
AI Poses Great Potential and Peril for Healthcare
At an Oct. 10 Conference, Experts from LDI and the Wharton Healthcare Analytics Lab Will Discuss the Thorniest Questions About AI
Blog Post
As artificial intelligence (AI) continues to reshape various industries, its impact on health care is particularly profound. In this blog post, we explore five key insights about the integration of AI technologies, including the promising role of large language models in clinical settings and the current challenges faced in their implementation. With expert perspectives from leaders, we’ll examine how AI is poised to enhance diagnostics, streamline workflows, and navigate regulatory hurdles in the coming years.
1. Large language models (LLMs) that record, transcribe, and summarize clinical notes are likely to be widely adopted within the next 10 years, predicted LDI Senior Fellow Hamsa Bastani, co-lead of the Wharton Healthcare Analytics Lab. The reason: Physicians are eager to have their workloads reduced. “We have a very clear business case for using these models,” Bastani said.
2. Currently, the most successful application of LLMs is happening in the field of radiology. Some large language models, can, for instance, interpret chest X-rays and write up the results. “That’s one place where tremendous progress has already been made,” said Bastani.
3. The FDA has now approved over 950 artificial intelligence-/machine-learning-enabled devices, 723 of which were reviewed by the radiology panel. However, “the FDA is approving them using a regulatory framework that was developed decades ago from non-AI devices. The rapid pace of technology development has outpaced the development of new, appropriate regulatory frameworks,” said LDI Senior Fellow Gary Weissman, Assistant Professor, Pulmonary and Critical Care Medicine, at Perelman.
4. The use of AI to diagnose illnesses has been hampered by the fact that when clinicians see patients face-to-face, they obtain more data than the large language models have available. For instance, a photo of a mole may not give as precise an impression as seeing one in real life. “The diagnostic tools are far from competing with humans because the models are not seeing the same information that physicians are,” Bastani said.
5. Clinical resistance is a big obstacle to the implementation and integration of AI functions. “Clinicians have a lot of expertise and authority, which makes changing the way they think and challenging their judgments particularly difficult,” said LDI Senior Fellow Marissa King, co-lead of the Wharton Healthcare Analytics Lab.
Register here for the LDI Wharton conference (Re)Writing the Future of Health Care With Generative AI on Oct. 10.
At an Oct. 10 Conference, Experts from LDI and the Wharton Healthcare Analytics Lab Will Discuss the Thorniest Questions About AI
First-of-Its-Kind National Analysis Shows Racial and Geographic Disparities
LDI Fellows Offer Specific Ways to Avoid Disparities and Serve Patients Better
Evidence from Philadelphia During the Pandemic Suggests Ways To Build Trust
Penn LDI Panel Unpacks Barriers and Options for Change
One Key May Be Medicaid’s Recent Coverage of the Routine Costs for Enrolling in Clinical Trials