Blog Post
How Machine Learning-Based Nudges Can Improve Cancer Care
Prognostic Tool Encourages Advance Care Planning
For patients with cancer, serious illness conversations (SICs)—structured conversations about prognosis, goals, and wishes—can reduce depression and anxiety, improve quality of life, and avoid unnecessary acute care utilization. Early SICs are now endorsed as a metric of high-quality cancer care. However, only 10% of patients with cancer have a documented conversation with their oncologist.
What’s preventing these conversations? One potential barrier lies with oncologists themselves, who may not estimate prognoses accurately. This is natural: oncologists are trained to focus on treating the cancer and giving a patient the best chance of surviving for as long as possible. When we are uncertain about the patient’s prognosis, we may be more likely to opt for aggressive treatment. Better tools to estimate prognosis could prompt oncologists to consider having discussions with patients and families about their goals and wishes before committing to aggressive therapy.
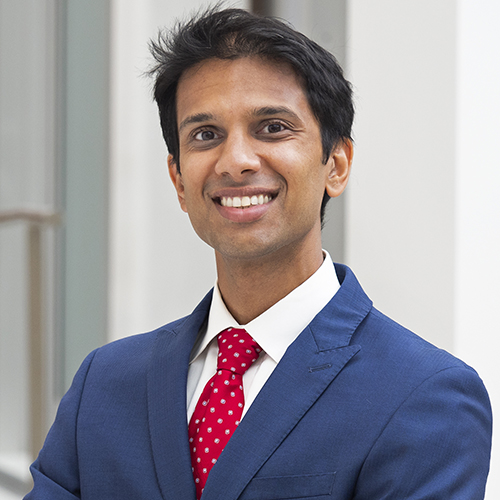
Machine learning could help. We previously developed and validated a machine learning algorithm to predict a patient’s risk of dying at 6 months. We found that the algorithm reliably distinguished between high- and low-risk patients and outperformed traditional tools in predicting mortality.
In a recent randomized controlled trial in JAMA Oncology, my colleagues and I studied the impact of an intervention based on this algorithm on rates of SICs among more than 14,000 patients seen in Penn’s Abramson Cancer Center. The intervention consisted of three parts. First, every week oncologists received an email about their performance on SICs and how they ranked compared to their peers. In the second part, oncologists could view lists of their upcoming patients who were identified as high-risk by the predictive algorithms. Oncologists could remove patients from the list who were not appropriate for a conversation at that time. In the third part, clinicians received reminder text messages on the morning of clinic for each patient identified as high-risk, prompting them to consider having and documenting a conversation in routine care.
We found that nearly 15% of high-risk patients in the intervention group had SICs, compared to 4% of high-risk patients in the control group. Even among patients who were not flagged by the text messages, rates of SICs nearly doubled. This suggests that our intervention had a “spillover” effect by encouraging doctors to think about having SICs with patients not on the high-risk list.
Of course, 100% of patients with cancer should have conversations about their goals and wishes; 15% is still much lower than where we need to be. Having these conversations is not only the right thing to do; it is a key component of many value-based care efforts in oncology. The Oncology Care Model—the only alternative payment model specific to oncology for Medicare beneficiaries—includes planning for end-of-life contingencies as a required component of a care management plan. Various other oncology organizations track rates of advance care planning internally as part of their quality metrics. Our intervention, which integrates health technology with behavioral nudges, could be a generalizable process to increase advance care planning among general populations with cancer.
The study, Effect of Integrating Machine Learning Mortality Estimates With Behavioral Nudges to Clinicians on Serious Illness Conversations Among Patients With Cancer, was published in JAMA Oncology on October 15, 2020. Authors include Christopher R. Manz, Ravi B. Parikh, Dylan S. Small, Chalanda N. Evans, Corey Chivers, Susan H. Regli, C. William Hanson, Justin E. Bekelman, Charles A. L. Rareshide, Nina O’Connor, Lynn M. Schuchter, Lawrence N. Shulman, and Mitesh S. Patel.