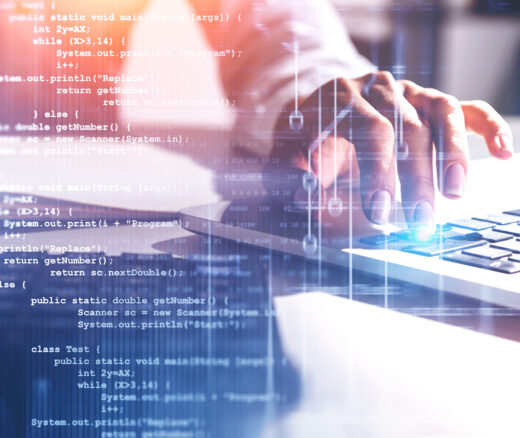
Health Care Algorithms Can Improve or Worsen Racial and Ethnic Disparities
Users Need To Understand Existing Disparities and Why Race Can Be Imprecise
Blog Post
Whenever we interact with a health care system, we generate a record. Increasingly, such records are digital in the U.S. and researchers use these electronic health records (EHRs) to study ways to improve care. For example, LDI Associate Fellow Emily Getzen and LDI Senior Fellow Qi Long use anonymized EHR data to build artificial intelligence/machine learning (AI/ML) models that predict health and disease in a population.
However, EHR data are often incomplete, creating a significant challenge for researchers. What’s more, data gaps may be unequally distributed across patient groups: People with less access to care, often people of color or with lower socioeconomic status, tend to have more incomplete EHRs.
Getzen and Long developed novel methods for better assessing the impact of incomplete EHR data on disease prediction—particularly the effect on model performance for Black and white patients. Their work discovered new potential consequences for health care inequities.
We asked Getzen and Long about their study.
We found that predictive models trained using incomplete EHR data performed poorly for patients with lower access to care. Our results were from extensive experiments using a new approach developed by our team.
A challenge to using real-world EHR data to directly assess the impact of incomplete EHR data is that we typically don’t know what and how much data are missing. We therefore simulated different amounts of realistic missing data in real-world EHR data to mimic varying levels of access to care. Our results suggest that the impact of missing EHR data is worse for Black patients than white patients. For example, in models that predicted future diagnoses of hypertension and diabetes, we saw strong disparities in predictive capabilities for Black patients.
Our results highlight the harmful consequences of using AI/ML models based on EHRs with biased incompleteness. If delivery systems and clinicians used such models to identify patients at risk for a condition or to evaluate their treatment, patients who are already in groups that are underserved could get left behind or even harmed by model inaccuracies about their predicted risk or health status. These models would perform poorly for underserved populations, propagating biases and exacerbating health inequities.
Some patients, such as immigrants or members of racially minoritized groups, may have difficulty getting care for a condition. Additionally, people with poor access may visit multiple clinics, so studies using data from a single EHR will miss some of their information. Health systems that serve disadvantaged communities also may not have the resources for EHRs.
Our work supports developing more equitable predictive models based on EHR data. We showed that current methods underestimate the adverse impact of incomplete EHR data on algorithmic fairness, that is, the ability of models to make predictions without bias. Our work demonstrates the need for better and fairer AI/ML models that achieve satisfactory performance for all, particularly people in underserved and disadvantaged groups.
For researchers, we demonstrated the importance of accounting for dependencies among medical events, such as lab tests and diagnoses, when assessing the impact of incomplete EHR data. Existing methods of model development often don’t account for dependencies among these events recorded in EHRs. An example is diabetes and insulin, which are related, so real-world EHRs either have or don’t have information on both items. Our new methodology provides a more accurate assessment of the inequitable impact of incomplete EHR data by accounting for these dependencies.
Our future work includes developing trustworthy statistical and ML models that can address potential biases from incomplete EHR data. We’re also interested in identifying effective strategies to reduce data incompleteness and improve the quality of EHR data for disadvantaged communities.
The study, Mining for Equitable Health: Assessing the Impact of Missing Data in Electronic Health Records, was published in The Journal of Biomedical Informatics on January 5, 2023. Authors include Emily Getzen, Lyle Ungar, Danielle Mowery, Xiaoqian Jiang, and Qi Long.
Users Need To Understand Existing Disparities and Why Race Can Be Imprecise
A Penn LDI Virtual Seminar Looks at Trends Driving the Crisis
José Escarce’s Presentation at LDI Lecture Series and Penn Medicine Health Equity Week Conference
Associate LDI Fellow Cited for Work with LGBTQ+ Youth
Receive Edward S. Cooper Award for Heart Safe Motherhood Project
LDI Researchers Develop A New Framework To Guide Health Systems