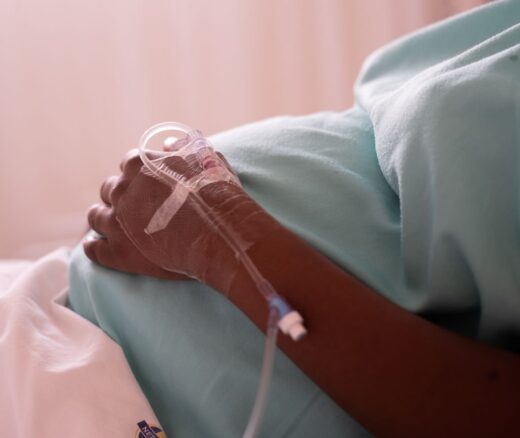
Over 500 U.S. Hospitals Have Stopped Delivering Babies Since 2010
A Crisis in Maternal Care is Unfolding—and it’s Hitting Rural and Urban Communities Alike
Blog Post
Travel in Greece was safer in the summer of 2020, thanks to a new approach to COVID-19 border screening developed by LDI Senior Fellow Hamsa Bastani and collaborators.
A new study in Nature demonstrates how Eva—a machine-learning system using up-to-date data on incoming travelers and their COVID-19 test results—helped Greece open for tourism while restricting entry of asymptomatic, infected travelers. The study found that Eva improved upon strategies that other countries used, including random testing of travelers at entry, or restricting travelers based on their country of origin.
Here’s how Eva worked: At least 24 hours before entry into Greece, travelers submitted a form reporting their demographics, origin country, and when they would arrive. Data from the forms, aggregated to remove individual information, were combined weekly with recent COVID-19 test results from travelers, also aggregated by characteristics such as age, gender, and origin country, including region of residence within that country to identify local hotspots.
The Eva algorithm estimated COVID-19 prevalence in incoming travelers, at a time when rapid tests were not widely available. Based on the predictions, some travelers were tested. Those with negative results entered the country and those with positive results quarantined. Eva used the results to continually improve over time via reinforcement learning, a type of machine learning. Each round of tests provided data to improve the next round of predictions.
In their new publication, Bastani and team analyze Eva’s effectiveness when it was used from August 6 to November 1, 2020 at all points of entry into Greece. During peak travel season, August to September, Eva processed data from nearly 42,000 traveler forms each day, directing nearly 17% of arriving households for COVID-19 testing.
Compared to the border strategy of randomly selecting travelers for testing at entry, during peak travel times, Eva helped Greece identify up to 4 times as many travelers with asymptomatic COVID-19 infections. Using Eva likely saved money: The researchers calculated that countries using random testing would have to administer 85% more tests to achieve Eva’s effectiveness.
In addition, the scientists compared Eva to the border control approaches used by most countries that depended on population-level reports of COVID-19 confirmed cases, deaths, or positive tests per capita. Compared to these typical approaches, Eva likely detected up to 1.45 times as many asymptomatic, infected travelers. Eva also provided information to Greece that policymakers combined with their own considerations, for example in deciding when to impose tighter restrictions, such as requiring a negative PCR test from anyone arriving from a specific country.
Bastani and the research team developed Eva as pro bono work for the Greek government. Realizing the ethical implications of collecting travelers’ data, the researchers designed Eva with policy and ethical considerations in mind. They met with lawyers, epidemiologists, and policymakers, to determine how detailed the data on travelers could be while ensuring individual privacy. As Bastani and colleagues note in a recent working paper, they emphasized the need for transparency in Eva’s design and algorithms, to build trust and buy-in among policymakers and public health experts in a period of global crisis. For efficiency and adaptability, the Eva design was modular, so parts could be updated, for example adding information about traveler vaccination status, without rewriting the entire system.
With Eva, Bastani and team showed that a reinforcement learning system, developed with attention to ethical application of its development and use, can identify more potentially asymptomatic, infected travelers than typical methods such as random testing or restricting entry based on population-level information. Because of the public health implications of less-efficient border screening methods, Bastani made the Eva code open source so countries can adopt and adapt the approach for their own borders.
The study, Efficient and Targeted COVID-19 Border Testing via Reinforcement Learning, was published in Nature on September 21, 2022. Authors include Hamsa Bastani, Kimon Drakopoulos, Vishal Gupta, Jon Vlachogiannis, Christos Hadjicristodoulou, Pagona Lagiou, Gkikas Magiorkinis, Dimitrios Paraskevis, and Sotirios Tsiodras.
A Crisis in Maternal Care is Unfolding—and it’s Hitting Rural and Urban Communities Alike
Stable Payments Improved Margins But Not Liquidity, New LDI Analysis Finds
LDI Senior Fellow Cited for “Significant Contributions” in Research
Outdated Laws Target Black and Queer Lives in Over 30 States, Fueling a Deadly Disease
Selected for Current and Future Research in the Science of Amputee Care
Research Memo: Delivered to House Speaker Mike Johnson and Majority Leader John Thune