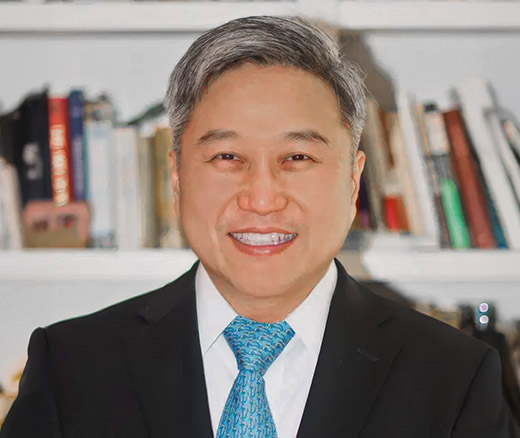
Hanming Fang Wins Best Article Award from Japanese Economic Review
Penn LDI Senior Fellow Paper Focused on the Mental Health of Older Chinese
News
As she opened the “(Re)Writing the Future of Health Care with Generative AI” conference, LDI Senior Fellow and Wharton School Health Care Management Professor Marissa King, PhD, pointed out that “there’s already immense penetration of generative artificial intelligence (AI) into healthcare and as we think about how we can harness it in order to improve the quality and efficiency of care, and reduce costs we must be mindful that these incredible possibilities come with a lot of risk. Our program today looks at how we can harness these possibilities while mitigating risk.”
In that same spirit of risk mitigation in the first panel, moderator Dan Gorenstein, LDI Adjunct Senior Fellow and the Executive Producer and Host of the Tradeoffs podcast, interviewed U.S. Department of Health and Human Services Acting Chief Artificial Intelligence Officer, Micky Tripathi, PhD, MPP, and pointed to the risk cited in an article published two weeks earlier in the New York Times. The piece detailed how 150,000 doctors and assistants at more than 150 health systems are using an AI feature in EPIC’s MyChart patient portal to draft their initial responses to patients. The Times pointed out how the “trend troubles some experts who worry that doctors may not be vigilant enough to catch potentially dangerous errors in medically significant messages drafted by A.I.”
Gorenstein further explained that, “A small study in the Lancet found that if left unedited, these messages would pose a risk of severe harm about 7% of the time.” He asked Tripathi to walk the audience through the federal approval process that a product like that MyChart AI feature has to go through before it’s released into the real world of patient care.
“There isn’t really a federal approval process it would have to go through,” said Tripathi. “It doesn’t qualify as a medical device by FDA definitions… And within electronic health record software, there’s no requirement that it go through some kind of approval process.”
Moderating the “Ethical Innovation: Patients, Doctors, and Large Language Models” panel, Ravi Parikh, MD, MPP, of Emory University School of Medicine, noted that the goal of the conference was to “expand a whole spectrum of AI hype and stories of positive implementation of large language models in health care to some more cautionary tales and aspects of regulation and how we can implement in careful ways.”
The panel addressed the complicated ethical challenges involved in the creation and use of LLMs for health care, particularly in relation to Predictive Decision Support Interventions (Predictive DSIs)—the AI-driven systems designed to assist in medical decision making by analyzing data and generating clinical predictions, classifications, or recommendations.
Panelist Maia Hightower, MD, MPH, the CEO and Co-Founder of Equality AI, an organization that provides AI quality assurance and compliance software for health care, emphasized the crucial importance of transparency, despite the enormous complexity of AI systems designed for health care purposes. She noted her strong support for the AI transparency regulations that the Department of Health and Human Services (HHS) Office of the National Coordinator for Health Information Technology (ONC) released in December.
That ONC transparency rule requires that health care AI developers provide detailed information on their algorithms, allowing healthcare providers to assess those systems’ fairness, appropriateness, validity, effectiveness, and safety. Developers must disclose information such as the source of data used for training, performance metrics, validation processes, and how risks associated with LLM outputs are managed.
“We do have this historical problem of clinical decision support tools being unregulated and not providing that level of transparency on where that data is coming from, how it performs across demographic groups, and whether or not health equity was taken into consideration,” Hightower said.
Because of the enormity of the planning, implementation, operation, and daily management and quality control of LLM-driven systems, health care systems are going to need a new layer of management headed by a Chief Health AI Officer, said panelist Julia Adler-Milstein, PhD, a Professor of Medicine at the University of California, San Francisco School of Medicine. Her institution has already created and filled that role as part of its AI governance process, supported by both a steering committee and a health AI oversight committee.
“It really is such a scope of work that we need a dedicated person to oversee it,” said Adler-Milstein who is the Director of the Center for Clinical Informatics and Improvement Research (CLIIR). “Our AI steering committee does something really important that hasn’t come up as much in today’s conference, which is talk about what problems are we trying to solve with AI. We almost walked into the room with the assumption that AI is good and doing good—but for what problems and what purposes? What are our health system’s biggest pain points? Which ones might best be solved by AI and how do we do a landscape assessment of all the tools that are out there to figure out if any of them look they’re ready for us to buy?”
“AI is often a mirror, and we don’t always like what we see when we look in the mirror,” said Sanmi Koyejo, PhD, Assistant Professor of Computer Science and Principal Investigator of the Stanford Trustworthy AI Research (STAIR). “But sometimes it’s actually useful when a mirror reflects to us some of the gaps in decision-making that individually seem rational, but collectively suggest bias of various kinds. A Stanford study evaluated a bunch of AI models and asked how they failed in ways related to equity. One quite interesting failure mode involved kidney failure and the EGFR measure that had a race correction and that was later fixed in the real-world literature and science, but not necessarily in the technology. When they evaluated AI models, the researchers found some still used the race correction.”
Asked what AI issue keeps him up at night, I. Glen Cohen, JD, Faculty Director of the Harvard University Petrie-Flom Center for Health Law, Policy, Biotechnology, and Bioethics, said it was knowing that “you can build an LLM system in such a way that it is scalable in terms of the AI, but are the review processes we’re talking about scalable? What happens when you are a safety net hospital and you too want the benefit for your population of these tools you’re hearing great things about? How do we know if the translation to this population is appropriate for the model? How can we do this ongoing review? And how can health systems share information without being sued for defamation when they are saying bad things about a model which produces terrible results for their patients?”
In the panel on how federal regulations relate to AI, Jenny Ma, JD, the Principal Deputy Director of the Office for Civil Rights at the U.S. Department of Health and Human Services, emphasized how the revised non-discrimination principles of the Affordable Care Act’s Section 1557 will, in July of 2025, be officially applied to health care AI. Section 1557 prohibits discrimination on the basis of race, color, national origin, sex, disability, age, or religion in activities that receive federal financial assistance, including health insurance plans, health care providers, health care facilities, and the AI systems they use.
“These non-discrimination principles are applicable to you if you are a covered entity that has violated 1557, whether you blame it on AI or not,” said Ma. “And we just wanted to affirm that.”
Jennifer Goldsack, MBA, Founder and CEO of the Digital Medicine Society (DiMe), a Boston-based non-profit focused on advancing the safe and equitable use of digital means to redefine and improve health care, emphasized why strong non-discrimination measures were needed in the health care AI field. She pointed to a recent investigative series called “Embedded Bias” in STAT News that detailed how race-based algorithms are already widely used throughout the health care delivery system and why it’s so difficult to change them.
DiMe has been partnering with the New York City Coalition to End Racism in Clinical Algorithms (CERCA) run by the New York City Department of Health and Mental Hygiene. Goldsack said the unusual thing about the project is how CERCA has gone across New York’s five boroughs talking with clinicians who are often able to identify discriminatory aspects of the algorithms responses they are already so familiar with from daily use.
“One of the things we’ve learned is that identifying these things is going to be very little about technology, and an awful lot about how we actually interact with the clinicians and administrators who use the AI. So, when we come to transparency, I want to say the notion that it’s purely a technological issue is not accurate,” said Goldsack. “It’s really interesting to think about how we can create a playbook to create incentives and scale CERCA’s approach and give a reason for every single public health department and every single health system to implement the same thing around the country.”
Penn LDI Senior Fellow Paper Focused on the Mental Health of Older Chinese
Incentives Worked Better than Culturally Tailored Videos, LDI Fellows Found
We Need Watchful Eyes at the Bedside, Two LDI Fellows Say
The Big Moneymaker is a Common Cardiac Surgery, Study by LDI Fellows Finds
Some Who Get Medicaid When Injured Have Longer Hospital Stays and Higher Costs, LDI Fellow’s Study Finds
Exploring Disparities, Hospitalization Outcomes, and Policy Solutions with LDI Fellow Nadir Yehya