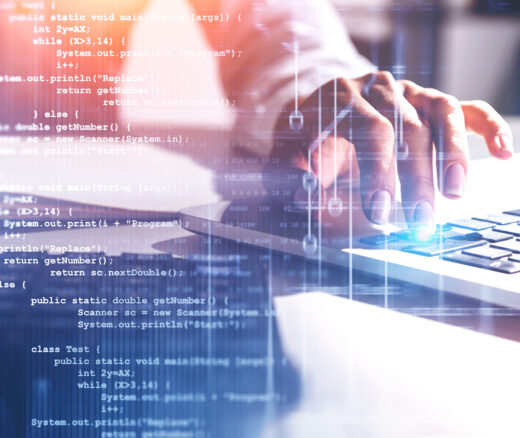
Health Care Algorithms Can Improve or Worsen Racial and Ethnic Disparities
Users Need To Understand Existing Disparities and Why Race Can Be Imprecise
Health Care Access & Coverage | Population Health
News | Video
Even as its development and hype explode ever outward, the case for what artificial intelligence’s (AI) clinical decision support role should or should not be remains obscured in tangles of unresolved legal, regulatory, ethical, and procedural issues. That was the central message of the January 12 University of Pennsylvania’s Leonard Davis Institute of Health Economics (LDI) virtual seminar that brought together four top national experts in the emerging field of medical AI.
The panel included I. Glenn Cohen, JD, Faculty Director of the Harvard Law School’s Center for Health Care Law Policy, Biotechnology and Bioethics; Rangita de Silva de Alwis, SJD, Director of the Global Institute for Human Rights at Penn’s Carey Law School; Nigam Shah, MBBS, PhD, Professor of Medicine and Biomedical Data Science at Stanford University; and Ravi Parikh, MD, MPP, Director of the Human-Algorithm Collaboration Lab at Penn’s Perelman School of Medicine.
Every aspect of the discussion underscored how AI’s dramatic rise is becoming—as de Silva de Alwis emphasized—the “Promethean moment of great promise and great peril” for both the field of medicine and the broader human society. The reference is to the legend of the ancient Greek god Prometheus who stole the technology of fire from the Gods on the Mount Olympus and gave it to mankind in the lowlands beyond, completely transforming human civilization.
“After a decade of hype,” said moderator Parikh as he opened the session, “the pace of how AI has evolved from tree-based predictive models and simple image-based classifiers to now generative text for clinical notes and diagnostic algorithms that rival the performance of a radiologist, or a pathologist has made us realize that AI has the potential to save lives and transform the practice of medicine. But there is also a lot of thinking that without guardrails, AI can disrupt care in harmful and inequitable ways.”
The panelists concurred that the implementation of AI in its various potential clinical formats will, in revolutionary ways, challenge drug regulation, medical device regulation, medical software regulation, the education of clinicians, the practice of medicine, the rights of patients, patterns of disparities, and medical tort law. There is currently no clear path through these intertwined challenges. Nor does there appear to be an emerging coherent vision on how best to address them all at the federal, state or industry level. In fact, that very fragmentation of the regulatory scene is itself an issue in the AI puzzle.
For instance, here’s a question that all state medicine boards may ultimately have to answer and define as a regulation: When ChatGPT starts giving you answers to questions about medicine, at what point does it start acting like a physician engaged in the practice of medicine versus acting like a computer program?
Calling clinically-related AI “an incredibly ethically and legally complicated space,” Harvard’s Cohen offered a list of some of the things regulators at all levels need to worry about, from the initial building of a clinical decision support algorithm model to its deployment in the service of patient care:
Cohen noted that the 21st Century Cures Act passed in 2016 to streamline and loosen approval requirements for the release of new drugs and medical devices would likely result in many AI medicine innovations not being reviewed by the Food and Drug Administration (FDA).
“All of this is tricky, but actually it gets worse,” Cohen said. “It would be silly to build an algorithm on data and then deploy it out in the world where there’s huge numbers of people and huge amounts of variation and tell that algorithm: ‘Don’t learn anything new. Stay with what you learn when you came out of the door.’ But we want algorithms to be adaptive and able to understand context and to learn as they go,” Cohen continued. “But that means that the ‘regulatory moment’ becomes much trickier now, because you can do as much advance review as you want, but when do you know whether it’s time for rereview? This is a very difficult problem. It is a problem much bigger than the FDA and even much bigger than the government regulation, because we have to worry about how private actors are self-regulating in this space.”
Shah pointed out that there are multiple federal government offices with jurisdiction over various aspects of clinical AI innovations and approvals at the FDA, the Office of the National Coordinator for Health Information Technology (ONC), the National Institute of Standards and Technology (NIST), and the Centers for Disease Control and Prevention (CDC). But he said that the only paradigm that gets much mention in the press or policymaking circles is the FDA’s Software as a Medical Device program, which has approved nearly 600 AI medical devices.
Parikh asked Shah to discuss the tradeoffs between regulation, overregulation, and the promotion of innovation in the market by allowing market forces, rather than policymakers, to dictate what makes it into clinical practice.
“That’s a tough question,” said Shah. “We like the freedom to experiment, but we don’t want to be experimenting with people’s lives and health outcomes. I think regulation should be injected around the actions you are taking with whatever AI technology you’re using. It would be a risk-based approach. If the action you’re taking is irreversible, like taking a lung or amputating a limb, we would want a very high bar for accuracy, quality assurance, and testing on that. On the other hand, if the action is relatively low risk—sending someone an email or ordering a Uber ride to an appointment—we can afford a little bit lower bar or threshold for experimentation.”
In another area of concern, Parikh noted that as AI systems gobble up vast amounts of data, they will inevitably ingest the biases that exist throughout those data troves. “Among the most prominent ethical issues raised by AI is its potential to bake in racial, ethnic, and gender inequities, and maybe inequities in subgroups that we’re not even thinking about,” he said.
De Silva de Alwis agreed. “My own work examines bias in the real world as well as in AI and as we know, data points are snapshots of the world we live in. They reveal real world biases on gender, race, ethnicity, disability, and intersectionality. No industry better illustrates this gap than the health care system,” she said. “For example, if AI systems are trained to recognize skin disease, such as melanoma based on images from people with white skin, they might fail to recognize disease of underrepresented populations. Second, if algorithms use health costs as a proxy for health needs, algorithms might falsely conclude that underrepresented minorities are healthier than their white counterparts.”
Another major issue that was of particular interest in questions submitted by the seminar audience was the question: “Who is liable when a clinical AI contributes to, or makes an error?”
“Essentially the liability rule is that if you follow the standard of care, even if it turns out there’s an adverse event, in theory you should not be liable for medical malpractice,” Cohen explained. “Part of the problem with AI is that it is particularly useful in cases where it’s telling a clinician to do something non-standard. This could be a patient who the AI predicts needs a different dosage or different approach. So, there could be a worry that liability concerns are causing you to disregard the AI precisely in the cases where it’s adding value.”
“Overall,” Cohen continued, “I think physicians are in a particularly bad position to be evaluating the quality of the AI they are using and to be making individualized decisions. The real state in my own view would be something more like enterprise liability for hospitals as purchasers of AI. So, the idea would be we’d have a set of rules about what is quality, and we’d expect hospital systems to be making determinations and really kicking the tires, and we’d have liability flow to the hospital systems if they fail to do so, or to the developers if they held stuff back.”
As the session ended, Parikh asked the panelists for a final comment on any AI-related issues that hadn’t been discussed but deserved further attention:
Shah: “We need a nationwide strategy to evaluate AI and assure that it’s meeting some minimum bar. Right now, there’s so much noise in terms of how to do bias control, how to do training and validation. We need a place where we can all go and test things on a large, diverse set of patient populations as opposed to just a one site or a few small cohorts—that would be a nationwide network of what we’ve called ‘assurance labs’ as part of the work on the Coalition for Health AI.
Cohen: “We haven’t talked much about generative AI or ChatGPT, but there’s a real hope that these may empower patients to practice the kinds of questions they might ask their physicians. But on the flip side, I’m terrified about the ease with which one can now create medical deepfakes, medical disinformation, and possibly fake records. So, I really think that this question of veracity of actual medical documents is something that we should keep an eye on.”
De Silva de Alwis: “The United Nations General Assembly acknowledged just last year that women are particularly affected by violations of rights to privacy in the digital age and the AI revolution and has called upon all states to develop preventative measures and remedies. It is important for human rights obligations to understand that states are largely defined by territorial sovereignty and territorial jurisdiction. But in the case of technology and AI, we must be able to pierce that territoriality to understand the extraterritoriality of companies such as OpenAI and Microsoft that have global reach and global power to do great good and to do great harm.”
Users Need To Understand Existing Disparities and Why Race Can Be Imprecise
A Penn LDI Virtual Seminar Looks at Trends Driving the Crisis
COVID-19’s Key Challenges Provide Insight for Future Outbreaks
Looking Forward, LDI Fellows Offer Their Perspectives and Expertise
Penn LDI’s Amol Navathe Testifies Before the U.S. Senate Committee on Finance
But More Funding and Better Rules Are Needed to Help People Who Are Addicted or Homeless, LDI Experts Say