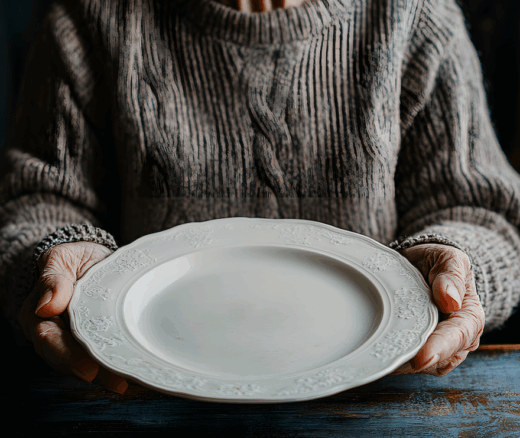
A Hidden Threat After Cancer: Food Insecurity and Early Death
Food-Insecure Cancer Survivors Live Shorter Lives, Especially if They Can’t Use Federal Programs Like SNAP
Blog Post
Artificial intelligence (AI) holds promise to advance mental health care, with potential applications including the use of social media data to predict depression and the use of chatbots to help fill gaps in the availability of therapists.
But are such technologies exempt from the racial disparities that plague other health care algorithms? And are the health care and technology worlds prepared to support the development of high quality tools that counteract disparities? Two new studies from LDI experts explore these questions.
In a recent study, Senior Fellow Sharath Chandra Guntuku and the Computational Social Listening Lab at Penn found evidence of significant differences in how people of different races speak about their depression. The investigators focused on language that is associated with depression, such as first-person pronoun use and negative emotion language, and they evaluated the relative performance of machine learning language models at predicting depression among Black and White Americans.
They found a relationship between greater depression severity and increased I-usage among White Americans but not Black Americans.
The investigators also showed that language-based prediction models performed poorly when tested on data from Black individuals, regardless of whether they had been trained using language from White or Black individuals. As a result, AI depression risk assessment from language data may not work for Black populations. In contrast, when such AI models were tested on White individuals, the tools showed relatively strong performance.
A second study by Guntuku and his team also found differences in how computer scientists and medical researchers evaluate AI mental health products. They reviewed medical and computer science research on conversational agents—i.e., chatbots and dialogue systems—designed to support conversations with people struggling with mental health. They found that computer scientists focus more on evaluating the response quality of chatbot responses with little emphasis on mental health application while medical professionals favor rule-based chatbots and outcome metrics to assess participants’ health outcomes—medical professionals also often relied on traditional technology to potentially favor better interpretability. The gaps between the priorities of the two disciplines led to poorer-quality tools in healthcare settings.
To learn more, we asked senior author Guntuku and his collaborators Sunny Rai and Jeffrey (Young Min) Cho a series of questions.
Rai: People tend to increasingly focus on self and negative thoughts with depression, and these patterns also manifest in the language that they use. For example, they may use more self-referential pronouns and express more negative emotions. With the use of sufficient language samples from individuals with depression versus individuals without depression, AI models can be trained to recognize changes in language linked to depression.
Rai: Knowing how language changes with depression can enable non-intrusive risk assessment for depression at scale. And, understanding how it varies with race will enable designing inclusive models.
AI predictive models for depression, in prior works, were trained and tested on users’ language on social media platforms such as Twitter and Reddit, which are known to have higher numbers of White adult male users. The question is, do such AI models generalize across races?
In this study, we focused on the most widely reported language markers: first person pronouns and negative emotions associated with depression. The next step would be to extend this study to other language markers to learn what indicates depression and for which races.
Rai: Language markers such as first-person singular pronouns and negative emotions are widely recognized markers of depression and have been identified in multiple previous studies. What this research shows is that the use of first-person singular pronouns may not be generalizable across races.
Rai: This needs to be researched further. One explanation is that depression does not manifest in the social media language of Black participants.
Guntuku: While our study found that Facebook language did not contain significant signals to identify depression among Black individuals, we do not know the exact reason why; it could also be due to variance in social media usage, self-disclosure on social media, and also the online vocabulary of depression. The challenge in obtaining further insight is to design research studies that account for representation among various racial groups. When the data collected is predominantly White, the resulting insights from such research may not be valid among other racial groups.
Guntuku: We need a tiered approach to utilizing predictive models or AI to detect health conditions. Digital data—including language, sensor, and images obtained after informed—consent could be used as a risk assessment measure, with the understanding that these are not diagnostic and will not replace a clinician. Individuals whose digital data shows elevated risk could be identified for a follow-up. Mental health challenges are increasing across the world and there is also an acute shortage of practitioners. AI—when developed, validated, and deployed in an ethical manner through multidisciplinary expertise and the involvement of all stakeholders—could play a vital role in bridging the diagnosis and treatment gap.
Rai: First, we need to specify the population characteristics in training and test data to avoid over-generalizing results that have yet to be tested on other populations. Second, we must aim for a racially diverse dataset, which is a more difficult task but that we must invest in to create inclusive models.
Rai: AI models for predicting behavioral health outcomes can lead to systemic health inequities if not tested and adjusted for diverse populations. This paper draws attention to the questions “Whom do these models serve?” and “How well?”
Guntuku: Any AI tools trained on data that is not inclusive would potentially fail to account for race-based differences.
Guntuku: We are building on this work to study differences in mental health experiences across cultures (beyond the U.S.) and to build a culturally sensitive large language model (LLM)-based conversational agent that can identify symptoms of depression and provide personalized support.
Guntuku: Beyond enhancing representativeness in data to train AI models in health care, we also need to investigate racial biases and promote transparency and accountability among companies and institutions that develop and use AI for mental health care to better allow for third-party audits of fairness and effectiveness.
Cho: More than a billion individuals globally are affected by various mental health disorders. Yet, a substantial number of these individuals lack adequate care. Financial constraints and limited availability of appointments contribute to this gap in health care provision. Mental health chatbots present one potentially feasible solution to these issues due to their cost-effectiveness and constant accessibility.
Cho: Computer science primarily concentrates on advancing the technical aspects of general-purpose chatbots rather than those tailored specifically for mental health. In contrast, the field of medicine tends to utilize traditional technology in health care chatbots, deliberately avoiding generative methods to maintain control over the dialogue. Additionally, computer science research often lacks human evaluation, leaving the impact on users unclear, whereas medical studies seldom perform automated evaluations on public datasets, which hampers the ability to compare different models effectively.
Cho: Given that interdisciplinary efforts make up only a small fraction of the work, we recommend increased collaboration to help close the gap between the two fields. We believe that both domains can mutually benefit and address their respective limitations through such partnerships.
The study, “Key Language Markers of Depression on Social Media Depend on Race,” was published on March 26, 2024 in Proceedings of the National Academy of Sciences. Authors include Sunny Rai, Elizabeth C. Stade, Salvatore Giorgi, Ashley Francisco, Lyle H. Ungar, Brenda Curtis, and Sharath C. Guntuku.
The study, “An Integrative Survey on Mental Health Conversational Agents to Bridge Computer Science and Medical Perspectives,” was published December, 2023 in Proceedings of the 2023 Conference on Empirical Methods in Natural Language Processing (EMNLP). Authors include Young Min Cho, Sunny Rai, Lyle H. Ungar, João Sedoc, and Sharath C. Guntuku.
Food-Insecure Cancer Survivors Live Shorter Lives, Especially if They Can’t Use Federal Programs Like SNAP
Outdated Laws Target Black and Queer Lives in Over 30 States, Fueling a Deadly Disease
Selected for Current and Future Research in the Science of Amputee Care
Penn LDI Seminar Details How Administrative Barriers, Subsidy Rollbacks, and Work Requirements Will Block Life-Saving Care
Top Experts Warn of Devastating Impact on Community Safety Efforts
A Gathering of a Health Services Research Community Currently Under Siege