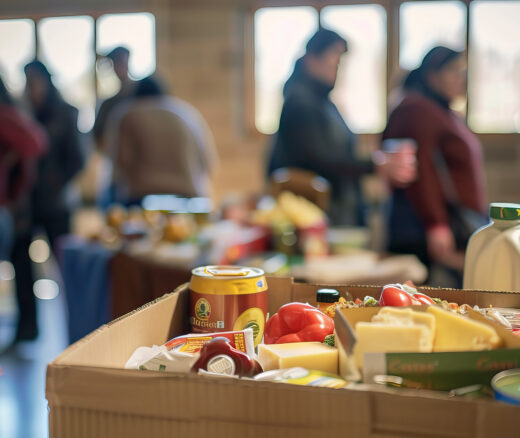
Parents Want Providers to Log Their Children’s Social Needs but with Transparency and Respect
Parents Also Fear Disclosing Social Needs Will Lead to Child Welfare Referrals, a Study by LDI Fellows Finds
Blog Post
In recent years, artificial intelligence has reached many parts of health care, and other health care algorithms are now part of everyday practice in clinical care, resource allocations, and health care management.
Diagnostic and predictive algorithms have become popular because they enable automation of common tasks and provide insight for difficult decisions. But many algorithms use race and ethnicity as input variables, raising concerns about their true predictive and diagnostic potentials.
At the request of the Agency for Healthcare Research & Quality (AHRQ), Senior Fellows Shazia Mehmood Siddique, Jaya Aysola, Michael O. Harhay, Harald Schmidt, Gary E. Weissman, and colleagues examined the impacts of health care algorithms on racial and ethnic disparities.
Their systematic review, involving 63 studies published since 2011, found evidence that health care algorithms can both improve and worsen racial and ethnic disparities for access, quality of care, and health outcomes for patients, regardless of their explicit inclusion of race or ethnicity as a variable.
Based on their findings, the investigators suggested strategies to reduce the likelihood that algorithms worsen disparities. These strategies include constructing algorithms with data that faithfully reflect diverse racial and ethnic groups and replacing existing race variables with more precise “non-race-based” variables, like genetic data or data on social determinants of health.
The research is timely as federal and state policymakers consider ways to protect the public against the unintended consequences of using predictive models and AI in health care.
For example, the Office of the National Coordinator for Health Information Technology within the Department of Health and Human Services recently finalized a rule that enacts transparency requirements for algorithms that Medicare and Medicaid electronic health records systems use for “predictive decision support interventions.” The goal of this new rule is to establish more clarity for clinicians about the design and performance of these decision support tools.
Congress is also discussing these issues, and both the Senate and House are considering legislation related to the accountability of AI and predictive algorithms. U.S. lawmakers in Congress are also striving to boost the public’s AI literacy.
We talked with first author Shazia Mehmood Siddique, MD, MSHP to learn more about the social impacts of health care algorithms and the crucial need for more policymaking:
Siddique: This study stemmed from a Congressional request: In 2020, several Congress members wrote a letter to the AHRQ detailing concerns about the use of race in clinical decision-making and requested a more comprehensive understanding of the impact of algorithms. AHRQ then released a request for proposal to conduct a systematic review, which was ultimately awarded to our team at Penn Medicine and ECRI.
Siddique: The addition of race into an algorithm can sometimes reduce racial and ethnic disparities, likely because race is utilized as the best proxy for the effects of systemic racism; this was shown in the revised Kidney Allocation System, and in a prostate cancer screening algorithm. However, race is ultimately an imprecise proxy variable, often used in place of genetic ancestry or complex environmental and social factors including social determinants of health. One risk of using race is that it can perpetuate the false notion that race is a biological construct; therefore it is important to transparently state what race is believed to be a proxy for and what efforts have been made to account for that in the existing dataset and algorithm.
Siddique: Yes, our review is just the tip of the iceberg. To make this work clinically applicable, we limited studies to only those that discuss racial and ethnic disparities in specific outcomes, like morbidity, mortality, timeliness of diagnosis, quality of care. However, there are many examples of algorithms—a notable one is Vaginal Birth after Cesarean Section (VBAC)—that primarily report on differential algorithmic performance across racial and ethnic groups without extrapolating to real-world clinical outcomes. While most equity experts believe that disparities are likely linked to use of the VBAC algorithm, this belief is often based on modeling studies and extrapolated data. This begs the question: how much evidence is needed before we call for change? The other issue is that racial and ethnic disparities are pervasive throughout medicine for a wide variety of reasons and attributing them to an algorithm is often challenging given existing study designs.
Siddique: It is important to use race and ethnicity intentionally and transparently in algorithms, and also to have an understanding of pre-existing disparities that algorithms may perpetuate before algorithm development and deployment. There is a need for more comprehensive data collection so that race’s proxy variables can be better identified and utilized, and also for incorporating diverse and multidisciplinary teams in algorithm development. I am currently working with the NIH to develop an ethical framework for the use of AI in biomedical and behavioral research—our report will be released soon!
Siddique: Our review examined the impact of algorithms on racial and ethnic disparities but did not look more broadly at the effect of clinical practice guidelines on racial and ethnic disparities. This is distinct because algorithms are mathematical formulas which calculate risk but there are many guideline recommendations that do not incorporate algorithms, but still call for race-based decision-making. We are now working on efforts to study how race and ethnicity are used in guidelines, examining the effect of existing professional society recommendations on disparities, and developing methods to mitigate racial and ethnic bias in clinical guideline development.
The study, “The Impact of Health Care Algorithms on Racial and Ethnic Disparities: A Systematic Review,” was published on March 12, 2024, in Annals of Internal Medicine. Authors include Shazia Mehmood Siddique, Kelley Tipton, Brian Leas, Christopher Jepson, Jaya Aysola, Jordana B. Cohen, Emilia Flores, Michael O. Harhay, Harald Schmidt, Gary E. Weissman, Julie Fricke, Jonathan R. Treadwell, and Nikhil K. Mull.
Parents Also Fear Disclosing Social Needs Will Lead to Child Welfare Referrals, a Study by LDI Fellows Finds
LDI Senior Fellow Lauren Eberly Leads Team in New Mexico
The U.S. Needs to Help Fix Migration’s Root Causes and Build Better Training Systems
A Penn LDI Virtual Seminar Looks at Trends Driving the Crisis
José Escarce’s Presentation at LDI Lecture Series and Penn Medicine Health Equity Week Conference
Associate LDI Fellow Cited for Work with LGBTQ+ Youth