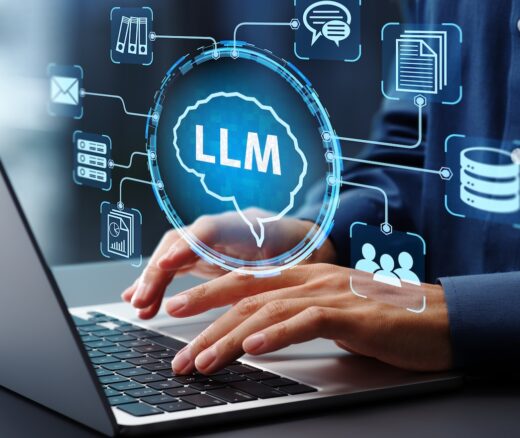
AI in Health Care and the FDA’s Blind Spot
A Novel Study Shows That Large Language Models Act Like Medical Devices in Clinical Care Scenarios
Blog Post | News | Video
Hospital emergency department (ED) triage practices—the rapid medical assessments that determine the severity of a patient’s condition and establish the priority of their treatment—have changed dramatically in recent years as EDs themselves have been revolutionized by new business and social dynamics. Constant overcrowding is now the daily norm in many EDs, with waiting room times dragging on for as long as eight hours.
One reason for this is that EDs are heavily impacted by the growing number of formerly multi-day in-patient treatments that are now performed as out-patient visits where patients with complications return to the ED. Another is that many hospitals face shortages of health care professionals, including doctors, nurses and support staff that limit their ED and inpatient treatment capacity. The rapidly expanding aging population whose members have more complex health issues is another driver of increased ED traffic. Primary care practices are also busier than ever, often with long waits and limited hours. And socioeconomic factors such as the lack of health insurance, underinsurance, and poverty also lead large numbers of people to EDs.
The Emergency Medical Treatment and Labor Act (EMTALA) requires that anyone who comes to an emergency department be given a triage examination to determine if they have an emergency medical condition. If such a condition exists, the hospital is required to provide treatment until the patient is stable. In theory, hospitals could screen patients in the ED, determine that no emergency condition is present, and refer patients to alternative services in a lower-cost setting, saving the health system, insurer, and patient money—and reducing ED crowding. In practice, this has rarely been attempted, in part because no algorithm exists that can safely determine who can be seen tomorrow and who needs to be seen today.
Researchers and insurers have long used one kind of algorithm to identify low acuity visits, though. These algorithms have been used in thousands of academic papers to see if improving other parts of the health care system could reduce low acuity ED visits. Improving these algorithms could offer a bridge to better future algorithms where patients are provided guidance to help them choose the best site of care, or to refer them to alternative sites of care when they reach the ED.
One of the most recent studies focused on this was conducted by a University of Pennsylvania team developing and testing what they call low acuity visit algorithms (LAVA).
“There’s been a call for more algorithmic approaches to emergency department triage but there are a lot of challenges to developing those algorithms,” said Ari Friedman, MD, PhD, who was one of the three-member Penn research team. “In this project we sought to develop a better algorithm for identifying low acuity emergency department visits. The current triage scoring system is incredibly widely used. There are about 4,000 papers we identified in previous work that use the existing approaches. And yet we show that in those approaches, if one of the existing algorithms declares an emergency department visit to be lower acuity, it turns out that there’s only a 1 in 3 chance or so that that visit was lower acuity by our definition. So, the existing algorithms leave a lot to be desired.”
Friedman, a Senior Fellow at the Leonard Davis Institute of Health Economics (LDI) and an Assistant Professor of Emergency Medicine at the Perelman School of Medicine, worked with two other team members: Angela Chen, MA, LDI Associate Fellow and MD/PhD student at the Perelman School and Wharton, and Richard Kuzma, MPP. Their paper, “Identifying Low Acuity Emergency Department Visits with a Machine Learning Approach: The Low Acuity Visit Algorithms (LAVA),” was published in Health Services Research (HSR), the health services research journal.
“In this study, we used national data on emergency department visits to try and determine if we could take the existing algorithms, which are essentially lists of diagnosis codes, and add in additional information to improve the reliability with which they detect visits that are lower acuity,” said Friedman. “We tried to test our hypothesis that a patient’s overall level of risk mattered a substantial amount, not just what happened on this particular visit. We ran the algorithm with only age and sex to try to predict who had a lower acuity visit and it turns out that giving the algorithm age and sex information is as good in performance as the existing algorithms using diagnostic codes. So that was our first real clue that we were on to something—it indicated that there was extra information available that the existing models weren’t using.”
“Then we took all the diagnosis codes previously created, added age and sex along with additional clinical information like, ‘Did you get a CAT scan?’, and also things available in claims data and the electronic health record and were able to improve performance by about 83%,” Friedman explained.
“In our algorithm, about two-thirds of the time when it labeled a visit as lower acuity, it was lower acuity by our definition. It’s important to note that identifying lower acuity two-thirds of the time is good and useful for some purposes but not very good for others, like insurers denying claims. One third of the time even as our algorithm labeled the visit low acuity, the patient was seen in the ED and an experienced clinician thought that patient needed at least two tests,” said Friedman.
“So, the findings of this study are that the existing tools, which are extensively used by academics and by insurers, have substantial room for improvement. Adding in more information about what happened while the patient was in the emergency department, what testing was ordered, as well as information about who the patient is, markedly improve the performance of these existing algorithms at identifying low acuity emergency department visits.”
Friedman pointed to three questions raises by the study’s methods and findings:
A Novel Study Shows That Large Language Models Act Like Medical Devices in Clinical Care Scenarios
A Penn Lab Takes a New Approach to Unpacking the Doctor-Patient Encounter
We Need a System That Watches AI Care at the Bedside, Two LDI Fellows Say
Machine-Learning Tool Combined with Behavioral Nudges Scaled Down Treatment While Maintaining Supportive Therapies
Oncologists Have Reservations About Prognostic Algorithms, but They Might Be Useful
An interview with Penn LDI Senior Fellow Hummy Song