Blog Post
Why It’s Hard to Evaluate State Policies in the Pandemic
Statisticians describe challenges in causal inference
As we speak, researchers are studying the efficacy of various state interventions in response to the Covid-19 pandemic. How different would deaths have been in New York if only schools were closed, while the rest of the economy remained open? How different would hospitalizations have been had California not locked down?
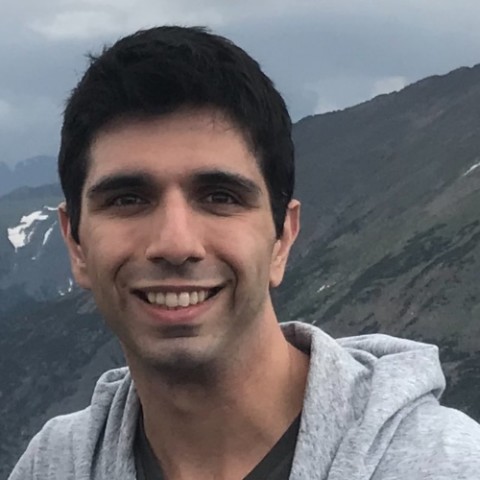
These questions are inherently causal, as they require a comparison of outcomes across “alternate realities” where everything but the policy intervention remains the same. Given that hopping between parallel universes is impossible (for now), statistical methods – such as matching, regression adjustment, inverse-probability weighting, difference-in-differences, and instrumental variables – rely on important assumptions. For instance, all causal methods rely on ignorability assumptions. Ignorability requires that after adjusting for various observed differences at, say, the state level, some other state that did not lock down is “comparable” to California. This allows us to estimate a counterfactual hospitalization rate that would have occurred in absence of lockdown using data from this comparable state.
However, achieving ignorability can be difficult. In the Covid-19 setting, residents have often been acting in anticipation of the intervention of interest. For instance, suspicion of an impending lockdown might spark a mass rush and crowding at supermarkets – increasing infection and subsequent hospitalizations. Yet, standard difference-in-differences analyses assume away these type of anticipation effects in the pre-period. In this setting, it may be difficult to find a comparable state that both did not lock down and had a similar mass rush in the pre-period. If we assign a comparator state that had no similar mass rush, we may very well find that this state had a lower post-intervention hospitalization rate. However, was this because the state had no lockdown or fewer mass interactions at supermarkets? It is difficult to tell with observed data.
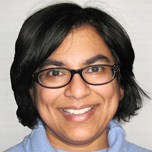
Suppose we can identify and measure all the relevant factors that drive differences in policy intervention and Covid-19 outcomes. It becomes difficult to actually find a non-intervention state where there is sufficient overlap of these factors as the intervention state. In causal terminology, this violates the positivity assumption. It leaves us with a bit of a catch-22. On one hand, we want to adjust for as many factors as we can to satisfy ignorability. However, the more factors we have, the harder it is to find control units comparable across this ever-growing set of factors. If we cannot find valid controls, we cannot estimate counterfactuals without imposing further strict assumptions.
Nearly all causal methods require non-interference – that interventions in one state, for instance, do not impact outcomes in another state. In the economics literature, interference is often referred to as “spillover” and is a certainty during pandemics. Consider that many of Hoboken, NJ’s residents work, socialize, and dine in NYC. It is obvious that a lockdown of NY impacts hospitalizations/infections in NJ in ways that are unclear. One could argue that Hoboken residents will substitute dining out in NYC with dining out locally, for instance. On the other hand, one could argue that Hoboken residents will socially distance as well. Either way, the policy choices of these states interfere with each other. Suppose the latter argument is true and that NJ does not lock down, yet exhibits low hospitalization rates. Is it because lockdowns are useless? Or is it because NJ residents began social distancing in response to NYC’s lockdown? It is very difficult to conclude either way using standard methods. This assumption also factors into individual-level analyses: if your close friends decide to socially distance, then that lowers your probability of hospitalization, even if you do nothing.
The consistency assumption underpinning causal methods also demands special thought in the Covid-19 setting. One of the ways in which consistency is violated is through non-adherence to interventions. For example, consider a state that does not encourage social distancing, yet residents actually practice social distancing anyway. Now suppose we see similar hospitalization rates relative to a comparable state that did encourage social distancing. If we do not consider consistency violations, we may falsely conclude that social distancing encouragement had no effect on hospitalization. Of course, the bias can run in either direction. In many states, adherence to lockdowns seems to be waning over time.
Causal considerations aside, we face many practical issues. States implement multiple interventions close in time: for example, implementing school closures and restaurant closures on the same day. This makes it difficult to disentangle the effects of each intervention from data. In addition, different states define lockdowns differently – requiring special care to make sure we have defined interventions consistently across units. Those wishing to use lockdowns as instrument in an instrumental variable (IV) analysis must contend with the fact that policy implementations are likely not random at all – but driven by many factors correlated with relevant outcomes – violating the exclusion restriction assumption underpinning IVs.
In conclusion, we advise researchers to proceed with caution when applying existing methods to analyze Covid-19 outcomes. This setting demands, more than ever, that we employ first-principles in assessing the validity of the underlying assumptions and take nothing for granted.