Blog Post
Leveraging Novel Real-Time Data for Getting Ahead of the COVID-19 Outbreak and Safely Easing Physical Distancing Policies
Since the U.S. federal government declared the novel Coronavirus (COVID-19) outbreak a national emergency, the impact has been varied widely across states, counties, and cities. It took New York City 10 days from the first case to reach 316 cases and close schools. Over the next 25 days the caseload exploded to 68,776 with reports of emergency medical services and hospitals stretched to capacity and rationing ventilators and critical care support. On the other hand, over 400 U.S. counties (13%) had yet to document their first case of COVID-19 as of April 16 when the White House released federal guidelines for reopening the economy.
To date, much of the policy and public health response to the novel coronavirus outbreak has been reactive and blunt — triggered by the number of COVID-19 cases or deaths in a given area — when it should be proactive and more precise based on a broader set of real-time data. State-level and hospital-level forecasting models can help plan mitigation strategies, but are limited by the reliability of the inputs to the models which are primarily COVID-19 cases and deaths and best guesses as to the degree of local physical distancing.
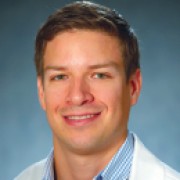
Given the still severely limited testing availability, waiting for a cluster of cases to appear is too late to get the maximum benefit of time-sensitive physical distancing policies and may misinform forecasting models. Further, emergency departments often do not test well-appearing patients who likely have COVID-19, so cases are likely vastly underestimated. Finally, the lag time from infection to illness to death makes the number of deaths a tardy signal to initiate aggressive physical distancing measures. Some U.S. states appear to be waiting for such a signal, with 5 states still having no stay-at-home orders in effect, and another 3 states have only partial orders covering only some cities or counties. The unreliability of COVID-19 case and death data and a poor understanding of the local degree of social distancing have led to dramatic shifts in predictions for given states in the most widely used model.
Novel indicators are needed to supplement COVID-19 case and death data and predict where testing, action, and resources are most needed. These indicators can also assess response to distancing policies and help inform when restrictions can be eased. Fortunately, a rapidly emerging set of new data sources, including smartphone location data and novel hospital-reported measures can meet this need. Ideal indicators would proactively and preemptively drive policy by revealing upstream or proximal signals about symptoms, movement, or contact, that could then predict cases before they are identified.
Smartphone-enabled surveillance and physical distancing levels
For example, a company that makes thermometers that pair with a smartphone app released a map of U.S. counties showing where fever measurements (potentially attributable to viral illness) exceed predicted fevers based on previous trends. The prediction model based on smart thermometer data was able to demonstrate an anomalous spike in febrile illness in Brooklyn starting March 1 and shortly after a similar spike in Florida, prior to when less than 200 COVID-19 cases had been diagnosed in each state. In addition to New York, Florida is now a hotspot of the epidemic with over 23,343 as of April 17. However, the map has also revealed a relative decrease in febrile illness rates in regions that have adopted distancing policies providing some early indications of policy effectiveness. In New York, a new app is now available that tracks symptom clusters using daily text messages.
Passively collected and anonymized smartphone location data, usually collected for advertising and marketing purposes, are yielding new insights on mobility and travel patterns and the potential for spread of the virus across states and countries. Striking mobility data visualizations have shown the travel patterns of individual smartphones identified on a Fort Lauderdale beach to cities and towns all over the northeast and midwest and smartphones in New York traveling all over the country and world. One company has aggregated smartphone mobility patterns at the state and county level to provide a distancing scoreboard. Google now provides state-level mobility trends stratified by types of trips (e.g., grocery, retail, workplace, transit station). These data could be used to measure the real-time impact of state physical distancing policies. Given the utility of these location data, governmental agencies are now partnering with these location data companies to inform policy.
Location-based smartphone applications can also be used to facilitate immediate “digital contact tracing.” There is new evidence that COVID-19 spreads too fast to be contained by manual tracing of contacts just prior to diagnosis. However, by integrating a contact tracing app into a phone operating system update, any individuals with the app active that were recently in close proximity to a newly diagnosed case could receive an immediate notification to self-quarantine and monitor symptoms. While contact tracing apps have been successfully used in Asian countries, they are not yet available in the U.S. Apple and Google have recently announced a partnership to embed an opt-in contact tracing app into their phone operating systems by May 2020.
Surveillance of local testing rates and population clusters
The first phase of the new federal plan to reopen the economy is a downtrend in new cases for at least 14 days. Several real-time maps show the total number of cases by state or county, but a new map by University of Chicago provides rates per 10,000 people, enabling clearer visualization of outbreaks occurring in rural areas such as southwestern Georgia, South Dakota, Navajo Nation, and Idaho. These areas have case counts per capita just behind New York and New Jersey. Embedded spatial models enable identification of both outlier case clusters and contiguous areas with relatively low activity. To re-open the economy, testing rates will need to dramatically increase. State-level data are now available and unfortunately testing rates nationally have plateaued over time when they should be increasing.
Hospital-based surveillance of illness and health care capacity
Emergency department (ED) encounter data can be used to monitor volumes of patients with syndromes consistent with COVID-19, building syndrome surveillance of influenza like illness. The CDC has just launched weekly ED surveillance reports for COVID19-like illness. Syndromic surveillance can capture spikes in patient illness that may be missed due to lack of testing and are available for select states.
Once cases of COVID-19 occur, surveillance of regional hospital capacity will be needed to coordinate interventions to address surge such as interhospital transfers, deployment of critical care resources such as additional staff, treatment sites, and ventilators. An important advance to meet this need is the CDC’s National Healthcare Safety Network (NHSN) new COVID-19 Patient Impact and Hospital Capacity Module. The Module enables hospitals to report daily counts of patients with suspected and confirmed COVID-19 diagnoses and current use and availability of hospital beds and mechanical ventilators. NHSN, in turn, will enable state and local health departments to gain immediate access to the COVID-19 data for hospitals in their jurisdictions. The COVID Tracking Project also reports total hospitalized and ICU patients in some states.
Implications for policy and data gaps
Participation in hospital-based surveillance systems such as the CDC NHSN is voluntary. The Centers for Medicare & Medicaid Services should provide financial incentives for participation.
While many of these hospital and smartphone data sources are available to the public as data visualizations (see Table), they will have the most potential to yield actionable insights if the levels of aggregation can be made granular enough for local decision makers to take action. Furthermore, raw deidentified datasets should be made open access to crowdsource new and rapid insights. This can be done while still maintaining individual and hospital privacy.
Table: Real Time Data Sources for COVID-19 Indicators
Data Element | Description | Level of aggregation | Sources | Public availability |
Illness indicators | ||||
COVID-19 tests | Counts of COVID-19 tests and % positive | State | COVID Tracking Project Johns Hopkins STAT | Data viz; Raw data |
COVID-19 cases and deaths | Counts of COVID-19 cases | State, county | UChicago Johns Hopkins NY Times STAT | Data viz; Raw data |
COVID-19 ED visits | Aggregate emergency department visit activity for COVID-19-like illness | Region and select states | CDC | Data viz; Raw data |
Atypical illness | Febrile illness from smartphone paired thermometers that exceed predicted levels of influenza-like illness | County | Kinsa | Data viz |
Predicted hospital use | Models that predict hospital use relative to hospital capacity | Hospital; State | UPenn (CHIME) UWashington (IHME) | Data viz |
Actual hospital capacity | Hospital self reported data on COVID-19 hospitalizations, ICU, and ventilator use and availability | Hospital | CDC National Health Care Safety Network | Data viz |
Social distancing indicators | ||||
Mobility | Mobile phone data tracking location change | State, county | Unacast | Data viz; raw data available to non-profits |
Mobility | Mobile phone data tracking location change | State | Data viz | |
Local mitigation strategies | Date of implementation of social distancing policy | State | AEI IHME | Raw data |
When digital contact tracing becomes available in Apple and Google smartphone operating systems in the U.S., federal oversight could ensure implementation that safeguards user privacy, similar to recent guidance passed in the European Union. For digital contact tracing to be successful, it estimated that 60% of the population would need to opt-in. Therefore, multiple policy efforts will be needed to nudge widespread adoption.
Many blindspots still exist where new data sources are needed. COVID-19 is disproportionately impacting and killing African Americans due to structural health inequities leading to overrepresentation in lower income communities that are less able to protect themselves with physical distancing and a higher susceptibility to serious illness due to higher baseline rates of chronic illness. All states should release death data by race and ethnicity as well as testing data by race to ensure that targeted mitigation efforts can be enacted and physical distancing policies can be sustained to reduce these disparities. Real-time data on hospital-level supplies of critical personal protective equipment (PPE) are also needed such as N95 respirators as well as critical medications. These fields could be added to the new CDC hospital capacity module. In addition to state and federal supplies, locations can request PPE donations via the #GetUsPPE website. Finally, there is an urgent need for a real-time COVID-19 national health information exchange database and a clinical registry for COVID-19 to describe the clinical characteristics of these patients, treatments received, and outcomes. This would catalyze rapid and actionable clinical and public health evidence while there is still time to make a difference given randomized trials results are not expected to make it into the peer review literature for months.
For the 400 U.S. counties with no reported COVID-19 cases yet, the many regions where case numbers are low but increasing, and regions where this disease is exacerbating existing minority health inequities, these novel data indicators can guide more precise and time-sensitive public health action to win the battle against COVID-19. Aggressive physical distancing measures are universally disruptive and we must ease them as soon as we can. However, we also must avoid the resurgence of cases that the latest models suggest we are likely to see when businesses and schools re-open.
To manage a return to “normal life”, we need data streams and predictive analytics that support contact tracing and other case-based containment strategies, as well as real-time assessments of herd immunity. The federal government would be wise to proceed with plans to integrate novel, tech-based COVID-19 indicators with existing CDC surveillance systems. Providing state-level dashboards showing testing data and death data stratified by race and ethnicity, case isolation, contact tracing and quarantining results (in addition to the symptom, mobility, health resource indicators described above) would allow local policymakers to equitably ease physical distancing restrictions with confidence.
When digital contact tracing becomes available in Apple and Google smartphone operating systems in the U.S., federal oversight could ensure implementation that safeguards user privacy, similar to recent guidance passed in the European Union. For digital contact tracing to be successful, it estimated that 60% of the population would need to opt-in. Therefore, multiple policy efforts will be needed to nudge widespread adoption.
Many blindspots still exist where new data sources are needed. COVID-19 is disproportionately impacting and killing African Americans due to structural health inequities leading to overrepresentation in lower income communities that are less able to protect themselves with physical distancing and a higher susceptibility to serious illness due to higher baseline rates of chronic illness. All states should release death data by race and ethnicity as well as testing data by race to ensure that targeted mitigation efforts can be enacted and physical distancing policies can be sustained to reduce these disparities. Real-time data on hospital-level supplies of critical personal protective equipment (PPE) are also needed such as N95 respirators as well as critical medications. These fields could be added to the new CDC hospital capacity module. In addition to state and federal supplies, locations can request PPE donations via the #GetUsPPE website. Finally, there is an urgent need for a real-time COVID-19 national health information exchange database and a clinical registry for COVID-19 to describe the clinical characteristics of these patients, treatments received, and outcomes. This would catalyze rapid and actionable clinical and public health evidence while there is still time to make a difference given randomized trials results are not expected to make it into the peer review literature for months.
For the 400 U.S. counties with no reported COVID-19 cases yet, the many regions where case numbers are low but increasing, and regions where this disease is exacerbating existing minority health inequities, these novel data indicators can guide more precise and time-sensitive public health action to win the battle against COVID-19. Aggressive physical distancing measures are universally disruptive and we must ease them as soon as we can. However, we also must avoid the resurgence of cases that the latest models suggest we are likely to see when businesses and schools re-open.
To manage a return to “normal life”, we need data streams and predictive analytics that support contact tracing and other case-based containment strategies, as well as real-time assessments of herd immunity. The federal government would be wise to proceed with plans to integrate novel, tech-based COVID-19 indicators with existing CDC surveillance systems. Providing state-level dashboards showing testing data and death data stratified by race and ethnicity, case isolation, contact tracing and quarantining results (in addition to the symptom, mobility, health resource indicators described above) would allow local policymakers to equitably ease physical distancing restrictions with confidence.