News
Report from a Penn Machine Learning Workshop for HSR Researchers
LDI-IBI Collaboration Triggered by Growing Faculty Interest
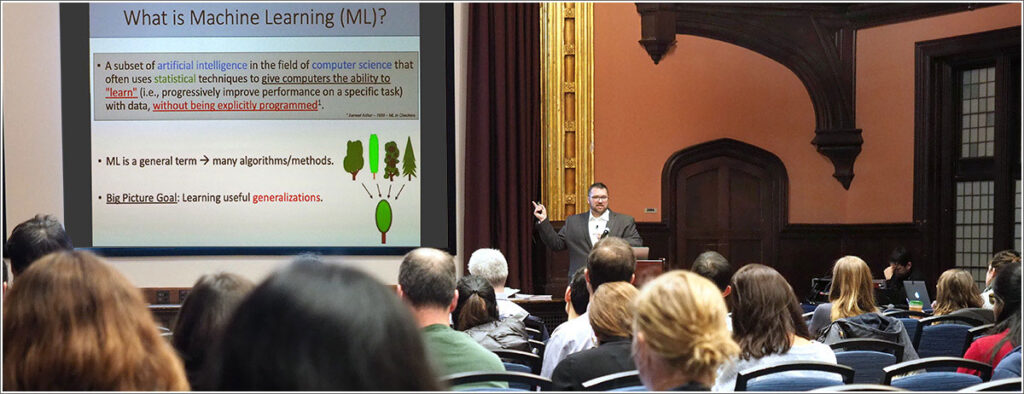
A two-hour workshop on the basics of how to apply machine learning methods to health care-related research drew an enthusiastic crowd of faculty and grad students to Penn’s Houston Hall. The majority of participants were from Penn’s Perelman, Wharton, Nursing, Arts and Sciences, and Law Schools, and the Children’s Hospital of Philadelphia (CHOP).
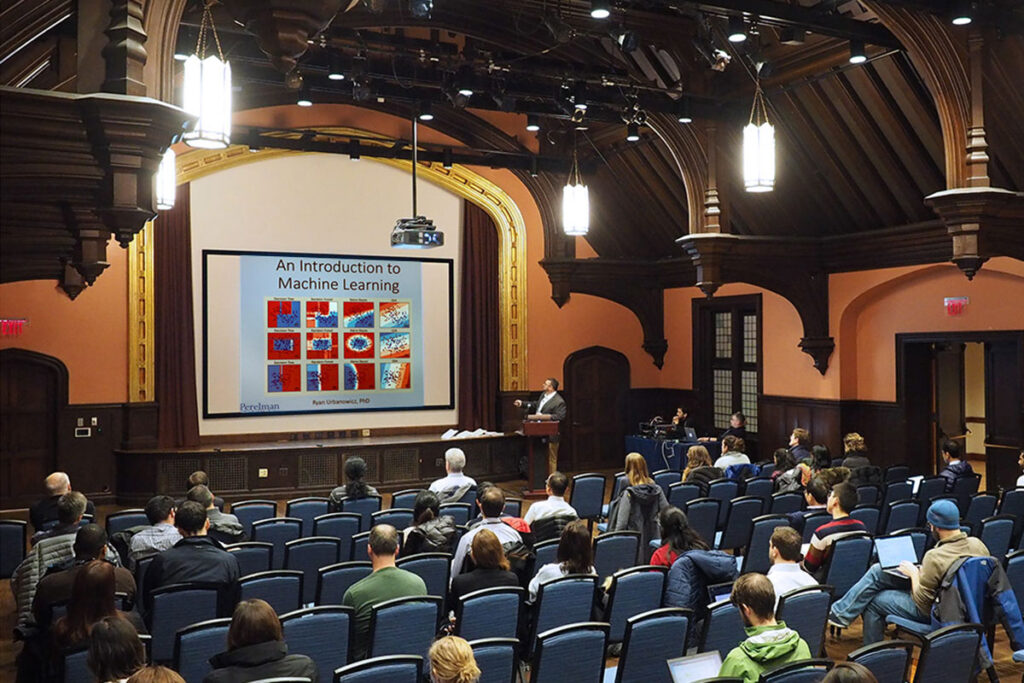
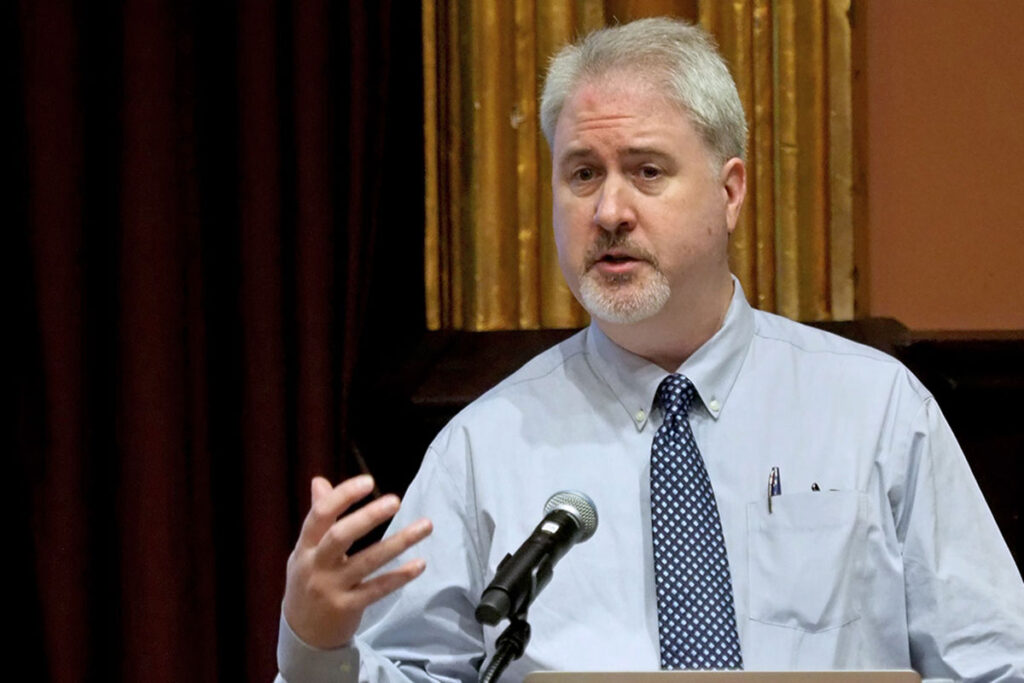
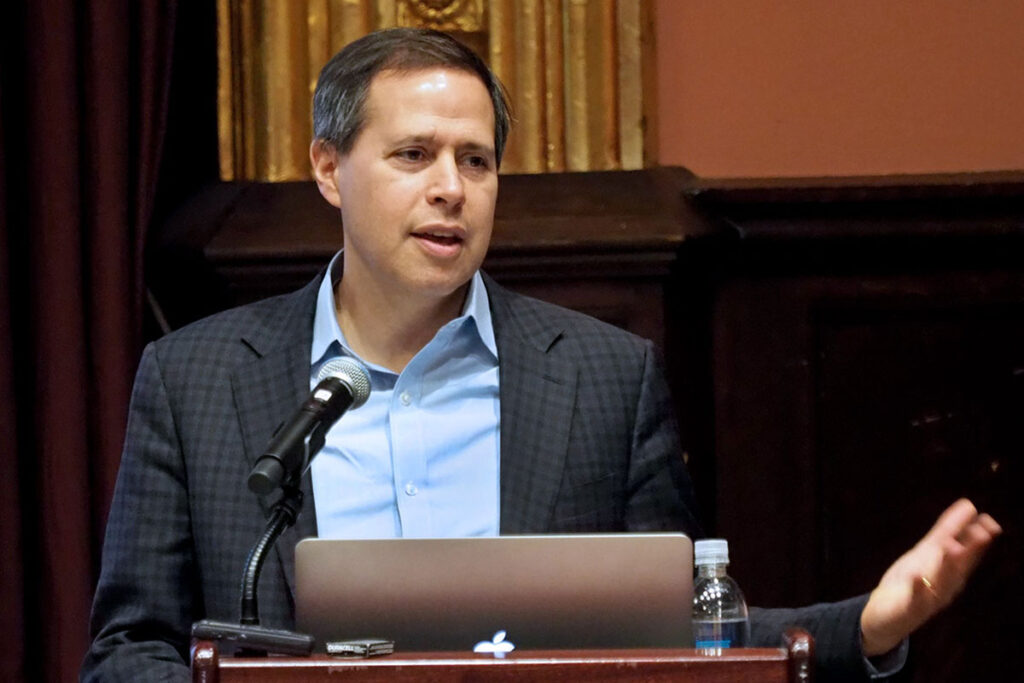
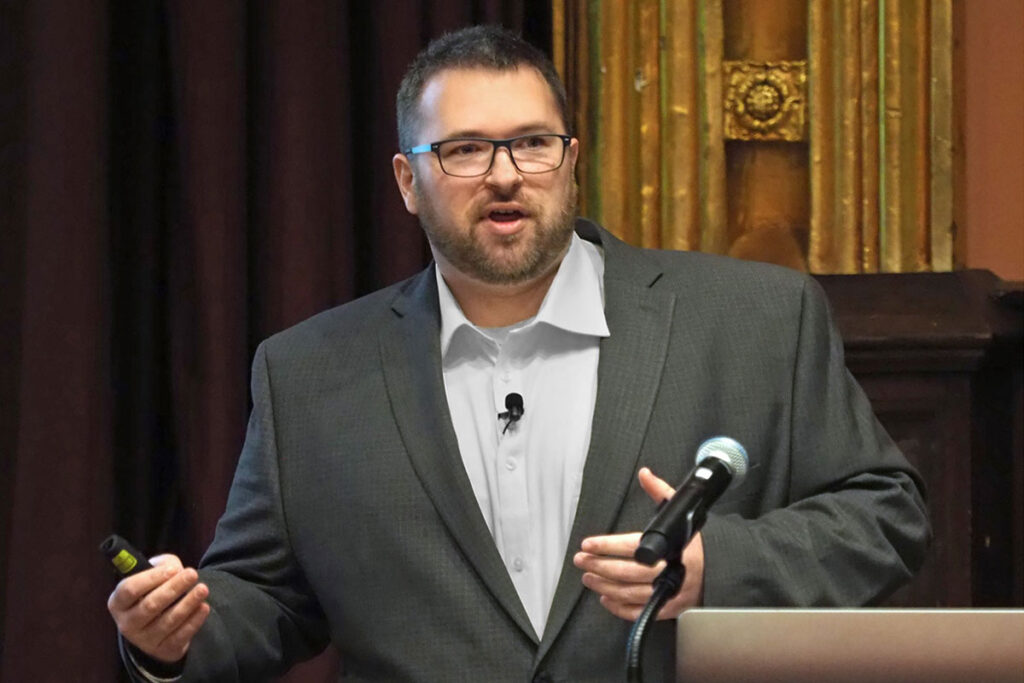
Nearly 170 faculty members and graduate students involved in health care research at the University of Pennsylvania flocked to the first machine learning workshop collaboratively organized by the Leonard Davis Institute of Health Economics (LDI) and Penn Medicine’s Institute for Biomedical Informatics (IBI).
In his opening remarks in the Gothic Revival auditorium of Penn’s Houston Hall, LDI Executive Director Daniel Polsky, PhD, cited the growing level of curiosity among health services researchers about the analytic capabilities of machine learning.
Growing interest
“In my own field — economics,” Polsky said, “a lot of very influential economists are now adopting machine learning techniques and writing really important papers. It triggered a growing interest among researchers trained in statistics or otherwise involved with big data sets.”
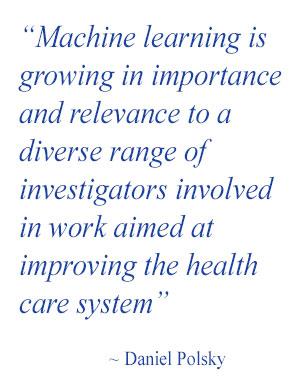
“The machine learning field is also clearly growing in importance and relevance to a diverse range of investigators involved in work aimed at improving the health care system,” Polsky continued. “This event kicks off LDI’s effort to draw this rapidly growing discipline into its collaborative mix for the benefit of our health services research Senior and Associate Fellows from across Penn’s schools.”
The move to embrace machine learning is in keeping with the other data-related research supports LDI already provides to its more than 400 Senior and Associate Fellows. These include the 13-year-old Health Services Research Data Center (HSRDC), housing a large collection of health care-related data sets, and the Health Economics Data Analyst Pool (HEDAP) of 20 masters- and PhD-level analysts and programmers.
“This is an important new discipline,” said Kevin Volpp, who helped organize the workshop, “because as we become more able to use large datasets and machine tools to examine questions that have previously been difficult to answer, we’ll be better able to understand how to design decision aids that offset some of the common decision errors humans make.” Volpp, MD, PhD, is the Director of Penn’s Center for Health Incentives and Behavioral Economics (CHIBE).
Predictive algorithms
A component of artificial intelligence, machine learning uses algorithms to analyze and assemble predictions from large data sets. The algorithms are able to make predictions about how to perform and continuously improve the performance of certain kinds of tasks without any outside input. In effect, the computer code system itself “learns” how to achieve better outcomes.
Now widely used in industry for things like detecting email spam or predicting the likely purchasing interests of online shoppers, machine learning has also been making significant inroads in both the biomedical and health services research side of U.S. health care.
It is now used by large health insurance companies for their chatbots, analysis of client data, management of hospital claims and as part of marketing research systems that identify and track new trends. It is also used to identify data anomalies that suggest health care-related fraud in areas like drug prescription billings. In yet another application, Johns Hopkins researchers are using machine learning techniques to improve outcomes of pancreatic cancer detection and treatment.
Recent machine learning papers
Just a few of the most recent papers published on the topic include:
- Machine Learning in Medical Imaging
- Machine-Learning Algorithms to Code Public Health Spending Accounts
- Predicting Patient-Reported Outcomes Following Hip and Knee Replacement Surgery Using Supervised Machine Learning
In his remarks to the audience, Jason Moore, PhD, Director of the Institute for Biomedical Informatics, said in recent years IBI has attracted a substantial membership of faculty scientists from across Penn schools “interested in using computational methods and technology for advancing health care.”
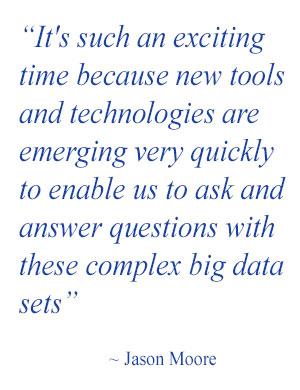
“We’re all enveloped with big data in our various disciplines,” he said, “and it’s such an exciting time because new tools and technologies are emerging very quickly to enable us to ask and answer questions with these complex big data sets.”
Education programs
Founded four years ago within the Perelman School of Medicine, IBI has built infrastructure and education programs that now involve 70 faculty members from Perelman and Penn’s Wharton, Nursing, Engineering, and Arts and Sciences schools.
The two-hour “Machine Learning 101” overview and discussion sessions were led by Ryan Urbanowicz, PhD, Assistant Professor of Informatics and Biostatistics at the Perelman School. (Download his set of 56 slides).
“Machine learning is about finding patterns or associations that can be used to make predictions,” Urbanowicz explained. “We start with some data, take an algorithm — there are many to choose from — to ‘train’ or generate a model that can take on many forms. Then we apply that model to make predictions on data we haven’t yet seen or data that we didn’t train the model with. That’s the goal of this process.”
Not a single method
“It’s important to understand that the phrase ‘machine learning’ is not referring to a single method. It refers to a whole family of methods offering different strategies,” said Urbanowicz, who is also the principle investigator at the newly launched Unbounded Research in Biomedical Systems Lab (URBS).
“The big picture of machine learning,” he said, “is that it identifies generalizable patterns in any given big data set.”