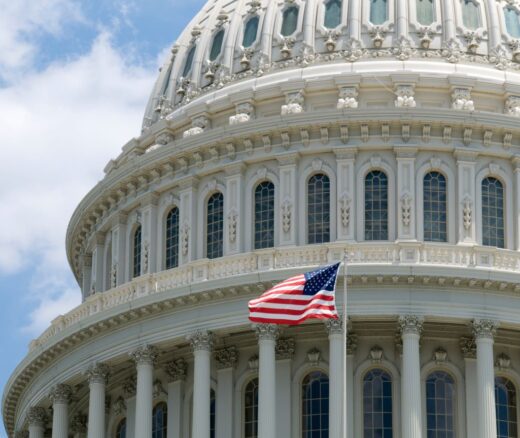
The Public Chafed the Last Time the GOP Tried to Cut Medicaid
Will This Time be Different? Past Health Bills Hold Clues
When patients with cancer and their oncologists talk about goals for treatment, it is called a serious illness conversation (SIC). These discussions can ensure that end-of-life treatments match patients’ wishes. Unfortunately, in many cases these conversations happen too late or not at all. Artificial intelligence(AI) can make it easier for oncologists to initiate these discussions.
Research by LDI Senior Fellow Ravi Parikh has shown that a machine-learning algorithm that identifies patients at higher risk of dying within a shorter time—in combination with behavioral nudges—can increase the rate at which doctors conduct SICs. Parikh built on that work in a New England Journal of Medicine AI study that examined how the machine learning-based behavioral intervention designed to motivate SICs affected health care spending at the end of life among patients who died.
By examining the last six months of hospital records of these patients, the researchers found that the intervention reduced the mean daily health care spending at the end of the patients’ lives. Among the 957 patients in the intervention group, the savings amounted to $13,747 for every patient who died, and more than $13 million in cumulative savings. The savings were the result of reduced chemotherapy, other cancer treatments, and office visits. Acute care, hospice, rehab, and long-term care expenses were similar for all patients, including those who were not in the intervention group.
Given that this is the one of the first studies to demonstrate that artificial intelligence—in this case, a machine-learning algorithm combined with behavioral nudges—is associated with reduced health care spending in cancer care, we discussed the findings with Dr. Parikh.
Parikh: I was surprised that the magnitude of savings was generally much larger than the impact on end-of-life chemotherapy rates. Since we knew that end-of-life chemotherapy had declined from our previous study, we suspected we would see savings, but the magnitude we observed was quite higher than expected. Of course, it is possible that we are observing savings that aren’t directly attributable to our intervention because there were a lot of things going on during the study period (like COVID). But given the strong savings that we observed pre- and post-SIC in the intervention group, I suspect that our intervention was a strong mediator of savings.
We also observed savings in the intervention group regardless of whether an SIC occurred. Chemo at the end of life declined even when there weren’t savings observed. It’s possible that our nudge may not have translated into a documented SIC, but still there was some impact on patient-centered decision-making that led to end-of-life savings.
Parikh: I think the benefit is less with machine learning/AI specifically, and more with applying a targeted approach to deploying large-scale but sometimes expensive behavioral interventions. When we use algorithms to target nudges, we can identify (phenotype) patients based on those most likely to respond and who often have high costs at baseline, often resulting in more change (delta) from a behavioral nudge to be had.
Another advantage of a machine-learning approach is a more granular and accurate risk stratification. Traditional behavioral phenotyping is based on isolated criteria and often doesn’t separate responders from nonresponders well. Machine-learning approaches, by using data-driven methods that incorporate many variables, can often improve assessment.
Parikh: Our study adds further evidence that scalable behavioral nudges plus data-driven risk stratification are an efficient way to target resources and save costs. Our study was done in a set of practices that weren’t uniformly participating in value-based payment, and as such there were still likely subtle incentives to give chemotherapy and perform more services. If this framework is embedded in a fully value-based practice or a practice transitioning to value-based payment, I would expect that there would be greater alignment of incentives to reduce unwanted and unnecessary services, perhaps by augmenting our intervention with ancillary care services like psychosocial counseling, after-hours symptom management, and evidence-based end-of-life care pathways that are pretty common in practices committed to value-based care.
Parikh: I see a lot of possibility for this framework in areas outside of end-of-life and palliative care, particularly those that require resources that promote shared decision-making interventions that are resource-constrained and require risk-based allocation. These include cancer screening navigation, decision-making around genetic testing, and care coordination to prevent hospital readmissions.
Parikh: I hope that these findings will spur health systems to make the necessary investments to integrate AI into existing care workflows, particularly by ensuring that they have modern software and data infrastructures to easily pull and push information into the electronic health record (EHR) or other electronic workflows.
Parikh: I think so. We need more prospective studies that show that we can embed AI into existing care workflows to enhance the right care, rather than framing AI as replacing oncologists, which I don’t think will ever happen in such a high-touch field like oncology.
Parikh: I see three big things happening:
1) Access to bigger, better data that will expand the availability of multimodal data from millions of patients to make AI models better. For our use case, this includes getting greater access to patient-reported symptoms or quality of life so we can build models that are centered on more patient-centric features and outcomes.
2) The incorporation of more conversational AI into clinical care so that patients can get more timely symptom management.
3) A move from prognostic modeling (e.g., predicting someone’s risk of death) to more predictive modeling, which will provide recommendations about whether to give—or not give—a particular treatment.
The study, “Spending Analysis of Machine Learning–Based Communication Nudges in Oncology,” was published on May 15, 2024 in the New England Journal of Medicine AI. Authors include Tej A. Patel, Jonathan Heintz, Jinbo Chen, Marc LaPergola, Warren B. Bilker, Mitesh S. Patel, Lily A. Arya, Manali I. Patel, Justin E. Bekelman, Christopher R. Manz, and Ravi B. Parikh.
Will This Time be Different? Past Health Bills Hold Clues
The Inflation-Adjusted NIH Budget is Lower in 2024 Than It Was at Its 2003 Peak
Supplement to Response to Request for Technical Assistance
But Many Other Factors Played a Role in Denying Loans, LDI Fellow Says
A Simple Change to Clinicians’ Prescribing System Reduced Prescription Disparities by Race, Ethnicity, Insurance Type, and Income
Offit and Buttenheim Criticize HHS Placebo Trial Mandate as Unethical, Misleading, and a Threat to Vaccine Confidence