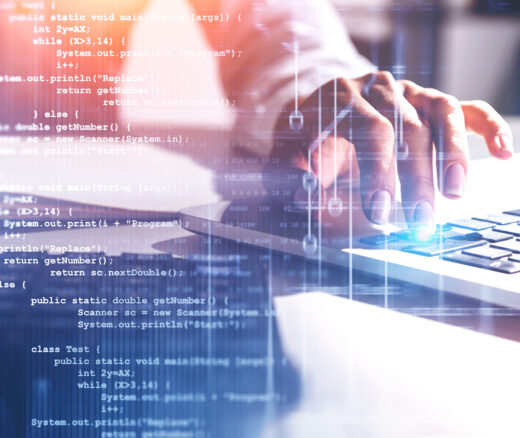
Health Care Algorithms Can Improve or Worsen Racial and Ethnic Disparities
Users Need To Understand Existing Disparities and Why Race Can Be Imprecise
News | Video
Although the idea of making artificial intelligence (AI) large language models full partners with clinicians in the diagnosis and treatment of patients is the most exciting potential for the innovative technology, the head of the just-launched Wharton Healthcare Analytics Lab (WHAL) emphasizes how caution will guide its work in this exploding new area of health services research.
“When you’re talking about AI and machine learning (ML) in health care, there are a great many challenges and potential unintended consequences,” explained LDI Senior Fellow Hamsa Bastani, PhD, a Co-Director of the new Wharton data initiative focused on health care delivery systems.
One of the goals of the new lab is to support the development of AI-based clinical decision support systems in which machines and humans work together as a true team in ways not yet completely tested or defined.
“We’re very excited about the potential use of large language models in a couple different ways,” said Bastani. “They can be trained on nursing notes and medical notes which are largely untapped sources of information and capture lots of useful information like socioeconomic factors, patient’s mental state and so on, that aren’t captured by traditional clinical features but do significantly affect health outcomes.”
She noted that such emerging algorithmic tools are not yet ready to be used directly on patients. “Right now, our focus is to ask, ‘Can they be clinician-facing with some supervision from the clinician?’ For example, the AI may draft medical notes or summaries, which a clinician could then look over and edit. That way, there’s a clear guard rail so we’re not letting these algorithms loose into the wild,” said Bastani.
Bastani has been deeply involved in AI and ML research since she earned degrees in Physics and Mathematics at Harvard and her PhD at Stanford University in 2017 before joining the faculty at the University of Pennsylvania. She has also been internationally involved in researching the use of algorithmic systems to optimize pharmaceutical supply chains in Sierra Leone, and COVID testing infrastructure in Greece. At Wharton, she teaches the Advances in Data-Driven Decision-Making course for PhD students.
Beyond clinical decision support, the WHAL that Bastani co-directs with her Wharton colleagues Marissa King, PhD, and Laura Zarrow, MSEd, will focus on algorithmic improvements in four other areas: resource allocation, workforce wellbeing, clinical trial practices, and health equity.
A chronic challenge in the area of health care clinical trials has long been the recruitment of enough participants as well as ensuring sufficient diversity among those participants.
“WHAL will be collaborating with Kevin Volpp, MD, PhD, and his team at the Center for Health Incentives and Behavioral Economics (CHIBE) to integrate dynamic adaptation and personalization into clinical trials,” said Bastani.
“Currently,” she continued, “clinical trials tend to be statically designed. They’re not actually personalized or dynamically customized in any way. We’ve been thinking about leveraging data from historical clinical trials or pilots to ‘warm start’ these predictive models. The collaboration with CHIBE gives us a very unique opportunity to do that, because they’ve been working on some of these problems for a long time. They’ve conducted numerous trials in the past and can leverage that data to learn something about patient response, and then leverage that information in a new clinical trial.”
CHIBE is involved in designing and testing behavioral interventions in a variety of different contexts working with health plans, employers, community-based organizations, and health systems. Volpp explained that, “In working with WHAL CHIBE is looking to develop new interventions that dynamically adapt, using AI to modify the intervention approach as each participant progresses based on what has been learned about the experience of other participants and that participant’s progress to date.”
One of the biggest concerns–and challenges–of AI and ML systems is that the data sets they train on are often laced with biases and those ingested biases become part of their logic and responses.
“The training data sets are very large, and so it’s not always feasible to debias the training data itself. But what we’re really interested in is the downstream decisions. For example, in our Sierra Leone work, we’re distributing essential medicines in some locations that have very low-quality data on the demand of different medicines. If we try to train a machine learning model on that, it will probably encode those biases and end up underserving locations that are already being underserved,” said Bastani.
“But there are many ways to address this, like using surrogate or proxy data sets,” Bastani continued. “In Sierra Leone, we might be able to use satellite data to infer population needs at a location, and this data is relatively divorced from the bias of the health system. So, by leveraging these auxiliary data sets, we may never perfectly debias the model, but at least the decisions that are actually coming out will be less biased.”
“A major issue related to algorithms, bias, and equity is that we often don’t have as high-quality data in our health systems for underserved populations or minorities, leading to worse health outcomes for minorities and underserved populations. That’s something that needs to be addressed. We’ve been thinking about leveraging proxy data there, perhaps with cheaper but more quantitatively available data like Google search terms,” Bastani said.
Another nonobvious issue already affecting the use of algorithm-driven devices in clinical work is the way in which many products don’t take into account the actual practices and workflows in places where they will be used.
“It’s crucial during the development of these systems that all stakeholders are brought together to ensure that whatever is designed and implemented actually serves all parties’ actual needs,” said Bastani. “We’re already seeing that a lot of the AI tools that have been approved by the Food and Drug Administration (FDA) aren’t being broadly used because they don’t really fit the clinician workflow effectively. Instead, they actually increase clinician workload.”
Users Need To Understand Existing Disparities and Why Race Can Be Imprecise
A Penn LDI Virtual Seminar Looks at Trends Driving the Crisis
COVID-19’s Key Challenges Provide Insight for Future Outbreaks
Looking Forward, LDI Fellows Offer Their Perspectives and Expertise
Penn LDI’s Amol Navathe Testifies Before the U.S. Senate Committee on Finance
But More Funding and Better Rules Are Needed to Help People Who Are Addicted or Homeless, LDI Experts Say