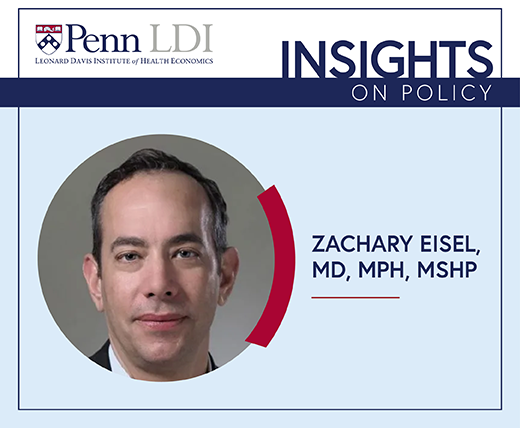
Estimated Overdose Deaths Due to the Loss of MOUD in the One Big Beautiful Bill Act
Research Memo: Delivered to House Speaker Mike Johnson and Majority Leader John Thune
News
The second annual J. Sanford “Sandy” Schwartz Memorial Grand Rounds event on June 8 was certainly in keeping with the kind of generational transmission the late Leonard Davis Institute of Health Economics (LDI) Senior Fellow and Perelman School of Medicine professor was known for. The event’s speaker was LDI Senior fellow Gary Weissman, MD, MSHP, a former Schwartz mentee. Weissman’s presentation, in turn, spotlighted the recent work of three of his own mentees who are engaged in various health equity investigations involving artificial intelligence (AI) or machine learning (ML) methods.
The gathering honored the memory and five decades of work of the late Schwartz, a highly respected and beloved Professor of Medicine and Health Care Management at the Perelman School of Medicine and The Wharton School. Schwartz, a former LDI Executive Director (1989-1998), died in 2021.
While his academic accomplishments and leadership positions were of the highest order, his most intense career passion was in guiding young scholars. That was the reason he won Penn’s highest honor for teaching. It’s also why this annual event honoring his memory was specifically designed to focus on today’s emerging young health services researchers.
“Being a mentor was one of the highlights of Sandy’s career, and he reveled in helping young researchers grow and become independent investigators with their own national reputations,” said Judith Long, MD, LDI Senior Fellow, Chief of the Division of General Internal Medicine at the Perelman School of Medicine, and former Schwartz mentee.
Event speaker Weissman recalled an evening 20 years ago when he was working as a carpenter’s assistant and was visiting Schwartz’s home as a friend of his children. Weissman spoke with the elder Schwartz, discussing computer modeling, and, to Weissman’s surprise, the professor ultimately offered him a job as a research assistant on a Penn computer modeling research project around breast cancer screening protocols.
“At the time, I thought I was just getting a job that was better suited to me than a carpenter’s assistant, but it turned out I acquired a mentor who became a lifelong friend. This was one of the main reasons I chose this career,” said Weissman, an Assistant Professor in Pulmonary and Critical Care Medicine in the Department of Medicine at the University of Pennsylvania Perelman School of Medicine. (Continues below photos)
Weissman pointed out that his recent search in PubMed found that Schwartz had collaborated with over a thousand different academics throughout his career. “That’s an enormous number,” said Weissman. “Most researchers are never that widely collaborative, and those numbers are actually an underestimate because they only include the papers Sandy was named on. There were a lot more downstream papers that Sandy wasn’t a part of but were fostered by him. His impact in this field was enormous.”
Weissman’s own presentation at the commemorative event was titled “Lighting the Way: Early Career Investigators, Advanced Methods, and Health Equity.” It reprised the current health disparities research work of three of his Penn mentees: LDI Associate Fellows Courtney Lee, MD, MPH; Alexander Moffett, MD, MSHP; and Jessica Lee, MD, MSHP.
~ ~ ~
Courtney Lee, a General Internal Medicine Fellow at the Perelman School of Medicine, and student in Perelman’s Master of Science in Health Policy Research (MSHP) program, is analyzing race-based linguistic differences in physician notes. The project began with a 2021 LDI pilot grant award. Based on text mining of electronic health records (EHR) systems using natural language processing, the research is exploring “testimonial injustice.” That’s when a clinician hears a patient describe their symptoms but doesn’t believe them because of their race or gender or some other reason that has nothing to do with the credibility of their statements. The clinician then uses “disbelief terms” in the patient notes establishing an “epistemic stance,” or how the clinician positions themselves relative to the credibility of a patient’s story.
“It’s the difference between reading that someone says: ‘Mrs. Smith has chest pain,’ or ‘Mrs. Smith reports chest pain,’ or ‘Mrs. Smith claims she has chest pain,’ or ‘Mrs. Smith denies she has chest pain,'” said Weissman, pointing out that these patient notes then go on to influence the understanding, attitudes, and responses of other clinicians engaged in Mrs. Smith’s treatment.
“‘Per the patient’ was another term being looked at,” said Weissman. “It’s a way of sounding smart because you’re using the Latin term ‘per’ but it’s really just a way of creating a feeling of distance from the patient’s story, like ‘supposedly, Mrs. Smith is having pain today.'”
“This entire line was Courtney’s research agenda,” continued Weissman. “It was a complex process that identified 40 some terms and boiled those down to a group of 13 that signify a disbelief that clinicians document in their notes.”
So far, in three hospitals the researchers have found significantly increased odds of Black patients having disbelief terms documented by physicians in their medical records.
~ ~ ~
Alexander Moffett, MD, MSHP, is an LDI Associate Fellow, an Instructor in Pulmonary, Allergy, and Critical Care at the Perelman School of Medicine, and, according to Weissman, a very adept computer technician. His research project is focused on algorithmic race corrections in the reference equations used to guide pulmonary function test interpretation such as those embedded by manufacturers in pulmonary function testing devices.
For several decades, the medical devices that report lung function contained a control equation that produced results that overestimated the lung capacity in Black patients—a measure based on traditional assumptions that Black peoples’ lungs were different from white peoples’. The result was that many Black patients failed to receive a diagnosis of a respiratory impairment. Or, when they did, the impairment was considered not as severe as that of an otherwise equivalent white patient.
Moffett looked at the question of what would actually happen if the reference equations were switched from the old set of race-corrected equations to a new set of race-neutral control equations published last year.
Tests of 5,000 white patients and nearly 3,000 Black patients, found that under the new equations, the odds of Black patients registering a respiratory impairment increased very substantially and the severity of the recognized impairments also increased substantially.
~ ~ ~
Jessica Lee, MD, MSHP, Adjunct Assistant Professor of Medicine at the Perelman School of Medicine, Medical Officer in the Division of Quality and Health Outcomes at the Center for Medicaid and CHIP Services, and former LDI Associate Fellow, is leading a research project analyzing the Food and Drug Administration’s (FDA) regulations for medical devices driven by artificial intelligence and machine learning AI/ML. The goal is to determine how the current regulatory framework for such a device might ensure equitable, safe, and effective functionality. This means ensuring that the device doesn’t produce racially disparate measures or outcomes. One recent example of this involves pulse oximeters that fit on a finger and measure blood oxygen saturation levels—a potentially critical diagnostic metric. The device was approved by the FDA but later found to perform differently among white patients and Black patients. The problem was that skin color significantly affected the reading—the darker the skin, the more inaccurate the measurement.
Weissman explained that under FDA rules that were developed many decades before AI/ML systems became widespread, such medical devices can be approved through the process of “substantial equivalence.” This means that manufacturers only have to demonstrate that their new device is equivalent to an older type of device that has already been approved. No further clinical evidence is required.
Currently, the FDA has about 500 AI/ML devices on its approved list. As Lee and Weissman began to assess the efficacy and equity of these devices, they selected 11 devices from the list that were relevant to critical care. They found that only one of the 11 had been approved via the FDA’s more rigorous “de novo” process involving clinical data and performance testing. They also found “no data whatsoever” about how the devices might perform differently among white and Black patients.
“This ‘substantial equivalence’ pathway may have worked for traditional medical devices, but AI/ML type medical devices are different, have different considerations, and we need different standards for them,” Weissman said.
~ ~ ~
Weissman’s own research heavily involves machine learning, AI, ML, social network analysis, natural language processing, and decision support systems required to develop informatics tools for the bedside.
As the Q&A session opened at the end of the presentation, audience member Peter Groeneveld, MD, MS, asked the first question addressing the implications of the AI conundrums apparent in the presented research projects.
Aside from being a physician, Groeneveld, an LDI Senior Fellow and Director of the Philadelphia Veterans Affairs Center for Health Equity Research and Promotion (CHERP), is also a Computer, Electrical, and Systems Engineer.
“Talk to us more about regulation of AI in the medical domain,” said Groeneveld. “AI is going to continue to wash over medicine in all kinds of ways we probably can’t even anticipate now. How in the world is the FDA—which is really designed to test drugs, hip replacement parts, and the like—going to keep up with the pace of innovation and protect patients and providers from the downside of this new technology?”
“There are probably two parts to the answer,” said Weissman. “One is the FDA has to keep innovating to figure out what the right regulatory model is. They started a few years ago with a precertification program for these devices but they closed that down last year because it wasn’t working well. The most recent guidance on clinical decision support from the FDA came out last fall and puts a lot of guardrails on devices. In my space in critical care, they made it pretty clear that any device that’s providing predictive clinical decision support in a time sensitive acute care context is going to be regulated—but they didn’t fully specify the nature or requirements for that regulation.”
“The other interesting angle,” continued Weissman, “is that the Office of the National Coordinator for Health Information Technology (ONC) is getting into this game. Just in the last couple weeks, the ONC released draft guidance on how they plan to regulate clinical decision support systems that are embedded in the EHR. The EHR was always their purview. It’s not clear to me if their guidance will apply to non-EHR based systems. Their approach requires much more transparency than anything the FDA has. And it’s been very responsive to the clinical AI community that’s also strongly focused on human factors and issues of trust.”
“In addition,” said Weissman, “the National Institute of Standards and Technology (NIST) also released an outline of how they think trust should be defined but I don’t think they’ve operationalized that into an instrument yet. But it is coming. So, I think those organizations need to also be involved to complement what the FDA is doing. But there’s still a million more miles of work to be done before that happens.”
Research Memo: Delivered to House Speaker Mike Johnson and Majority Leader John Thune
Research Memo: Delivered to House Speaker Mike Johnson and Majority Leader John Thune
Hospitals’ Current Cost Reports are Unreliable and Should be Improved, LDI Fellow Finds
If Federal Support Falls, States May Slash Home- and Community-Based Services — Pushing Vulnerable Americans Into Nursing Homes They Don’t Want or Need
Historic Coverage Loss Could Cause Over 51,000 People to Lose Their Lives Each Year, New Analysis Finds
Cited for “Breaking New Ground” in the Field of Hospital Operations