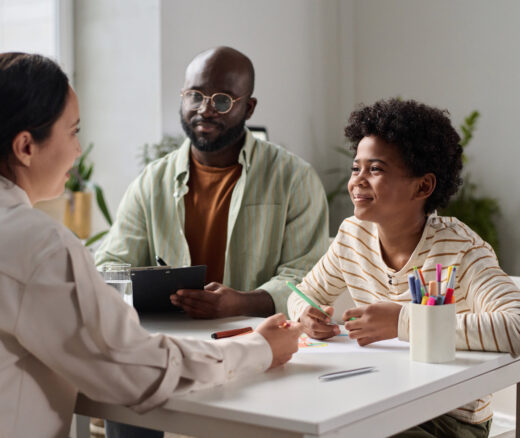
Is There a Role for Pediatricians in “The Talk”?
A Penn LDI Study Provides Insights on the Challenges and Opportunities of Addressing Police Encounters With Their Patients
News
The LDI-Penn Medicine Research Laboratory is a strategic granting partnership designed to advance LDI’s mission of developing generalizable health care knowledge for the nation and to generate actionable knowledge which guides Penn Medicine’s operational decisions towards ensuring that patients receive the best care. Its 2025 grant award proposals focused on “Leveraging Technology to Improve Health Care Delivery.” Below are the two winners.
Principal Investigator: Eileen Lake, PhD, RN | Penn School of Nursing
The use of electronic health records (EHRs) in clinical workflow permits the understanding of patient care elements that were previously hidden. Nurses constantly surveil whether patients needing a higher level of care or not. Missed care is not having relevant surveillance. Nurses’ vital sign frequency and free text nursing documentation are EHR clinical workflows that have been linked to patient deterioration. Unexpected intensive care unit (ICU) transfer (from a non-procedure unit) is an episode of critical patient deterioration that if warranted, reduces patient morbidity and mortality. Racial biases in surveillance, prediction, and transfer may occur, yielding inequitable care, unreliable and invalid predictive models, and inequitable patient outcomes, which present a research imperative. There is no published literature about patient demographic bias in nursing surveillance and unexpected ICU transfer, making the project novel. The study objectives are to utilize machine learning to predict unexpected ICU transfer from nursing documentation and determine if inclusion of demographic variables improves model performance and reflects missed surveillance of racial minorities and non-English primary language patients. The design is a retrospective cross-sectional study of one year of surveillance and patient outcome data (2023) from the Hospital of the University of Pennsylvania (HUP) and Penn Presbyterian to provide variation in the mix of patients, nurses, attending physicians, and other caregivers. This application focuses on improving care efficiency at Penn Medicine through optimizing technology, including the EHR, while preserving and advancing the quality, safety, and equity of care. Current screening tools do not capture patient race/ethnicity. This structural inequity in the prediction of patient deterioration will be addressed in the proposed project.
Principal Investigator: Eric Bressman, MD, MSHP | Perelman School of Medicine
Co-Investigators: Subha Airan-Javia, MD; Thomas Savage, MD; and Kaustav Shah, MD, all of the Perelman School of Medicine
Patient handoffs are an important and necessary step when transitioning care between providers, however there are often communication failures that occur during handoffs that contribute to medical errors and composing handoff contributes to providers’ already large documentation burden. Large language models (LLMs) and other generative artificial intelligence (AI) solutions can help improve documentation by automating the ability to create understandable text and constantly updating information from various sources in the medical record. LLM-generated clinical summaries have shown comparable performance to clinicians for certain tasks, but have not been studied in handoff-related tasks. Specific aims include: 1) Implement and train an LLM-based tool that will generate end-of-rotation handoff and evaluate its performance; 2) Study the impact of the LLM-generated end-of-rotation handoff on documentation burden and work exhaustion in a randomized controlled trial of inpatient hospital medicine providers. Research Plan: To accomplish these aims, we will first use an iterative training process including prompt engineering and few-shot learning to optimize LLM output for end-of-rotation handoff, integrate the feature into CareAlign, and compare handoffs generated by the LLM and clinicians using blinded reviewers on domains of quality and errors. Next, we will conduct a randomized controlled trial where inpatient general medicine attendings will be randomized to have access to the LLM feature to generate an end-of-rotation handoff. Measures of documentation burden and work exhaustion will be surveyed in both groups along with the quality of the handoffs from both the providers composing and receiving the handoff.
A Penn LDI Study Provides Insights on the Challenges and Opportunities of Addressing Police Encounters With Their Patients
Chief Transformation Officer Raina Merchant Provides Overview and Insights at LDI Annual Leighton Memorial Lecture
LDI Researchers Analyzed Ethnicity, Race, and Sex‐Specific Population Participation in Clinical Trials
As Congress Considers Cuts to Medicaid Spending, Policymakers Should Better Account for States’ Ability to Pay
Bead-Filled Bottles May Change Attitudes and Help Prevent HIV Transmission, LDI Fellows Show in Philadelphia Studies
Penn LDI Researcher Derek Griffith’s Project Unpacks the Process